Hong Kong Med J 2024 Aug;30(4):271–80 | Epub 25 Jul 2024
© Hong Kong Academy of Medicine. CC BY-NC-ND 4.0
ORIGINAL ARTICLE CME
Diagnostic accuracy of a prehospital electrocardiogram rule-based algorithm for ST-elevation myocardial infarction: results from a population-wide project
Joanne HY Lai, MB, BS, FHKAM (Emergency Medicine)1; CT Lui, MB, BS, FHKAM (Emergency Medicine)1; Total WT Chan, MB, ChB, FHKAM (Emergency Medicine)2; Ben CP Wong, MB, ChB, FHKAM (Emergency Medicine)2; Matthew SH Tsui, MB, BS, FHKAM (Emergency Medicine)3; Ben KA Wan, MB, BS, FHKAM (Emergency Medicine)4,5; KL Mok, MB, BS, FHKAM (Emergency Medicine)4,5
1 Department of Accident and Emergency, Tuen Mun Hospital, Hong Kong SAR, China
2 Department of Accident and Emergency, Tin Shui Wai Hospital, Hong Kong SAR, China
3 Department of Accident and Emergency, Queen Mary Hospital, Hong Kong SAR, China
4 Department of Accident and Emergency, Ruttonjee & Tang Shiu Kin Hospitals, Hong Kong SAR, China
5 Fire Services Department, Hong Kong SAR, China
Corresponding author: Dr Joanne HY Lai (joannelaihy@fellow.hkam.hk)

Abstract
Introduction: This study reviewed the diagnostic
accuracy of the prehospital electrocardiogram
(PHECG) rule-based algorithm for ST-elevation
myocardial infarction (STEMI) universally utilised
in Hong Kong.
Methods: This prospective observational study
was linked to a population-wide project. We
analysed 2210 PHECGs performed on patients who
presented to the emergency medical service (EMS)
with chest pain from 1 October to 31 December
2021. The diagnostic accuracy of the adopted rule-based
algorithm, the Hannover Electrocardiogram
System, was evaluated using the adjudicated blinded
rating by two investigators as the primary reference
standard. Diagnostic accuracy was also evaluated
using the attending emergency physician’s diagnosis
and the diagnosis on hospital discharge as secondary
reference standards.
Results: The prevalence of STEMI was 5.1%
(95% confidence interval [CI]=4.2%-6.1%). Using
the adjudicated blinded rating by investigators as
the reference standard, the rule-based PHECG
algorithm had a sensitivity of 94.6% (95% CI=88.2%-97.8%), specificity of 87.9% (95% CI=86.4%-89.2%),
positive predictive value of 29.4% (95% CI=24.8%-34.4%), and negative predictive value of 99.7%
(95% CI=99.3%-99.9%) [all P<0.05].
Conclusion: The rule-based PHECG algorithm that
is widely used in Hong Kong demonstrated high
sensitivity and fair specificity for the diagnosis of STEMI.
New knowledge added by this study
- The prehospital electrocardiogram (PHECG) diagnostic algorithm universally utilised in Hong Kong had high sensitivity for diagnosing ST-elevation myocardial infarction (STEMI) in a population-wide cohort of patients with chest pain.
- One in eight ECGs showed false-positive results for STEMI; the leading causes were early repolarisation, left bundle branch block, and extreme tachycardia.
- Evolving ECG patterns, subtle ST-segment elevation, and STEMI equivalents were responsible for false-negative diagnoses.
- Primary diversion of STEMI patients to centres capable of primary percutaneous coronary intervention should not be implemented solely based on the algorithm’s ECG diagnosis.
- ST-elevation myocardial infarction can be reasonably excluded by the PHECG diagnostic algorithm.
- Physicians should be aware of STEMI equivalents that are not identified by the algorithm.
Introduction
Heart disease is the third leading cause of death in
Hong Kong. In 2019, an average of approximately 10.2
people died from coronary heart disease each day.1
International guidelines recommend prehospital 12-lead electrocardiogram (ECG) for the assessment
of patients with suspected acute coronary syndrome
who present to emergency medical services
(EMS).2 3 Prehospital triage with direct transfer to
the cardiac catheterisation laboratory for primary percutaneous coronary intervention is a strategy
adopted by various healthcare systems to reduce
reperfusion time in patients with ST-elevation
myocardial infarction (STEMI).4 5 Previous studies
have investigated the diagnostic performances
of prehospital electrocardiograms (PHECGs)
for STEMI by various automated algorithms,6 7 8 9 10
trained onsite EMS personnel,11 12 and emergency
department (ED) physicians remotely interpreting
the tele-transmitted ECGs13; the findings have
implications for policymakers involved in planning
systems of care to minimise inappropriate resource
mobilisation.
In Hong Kong, the Hospital Authority, the
local public healthcare service, and Hong Kong
Fire Services Department, the primary EMS
provider, jointly launched the Prehospital 12-Lead
Electrocardiogram for Chest Pain Protocol on 1
February 2021. The Protocol covers the catchment
areas of all EDs in Hong Kong and serves a population
of 7.41 million. This study utilised data from a
territory-wide audit of the Protocol to determine the
diagnostic performance of the PHECG algorithm for
STEMI.
Methods
Study design and setting
This prospective observational study analysed data from the territory-wide audit project regarding the Prehospital 12-Lead Electrocardiogram for Chest
Pain Protocol, led by the Hong Kong Hospital
Authority Coordinating Committee in Accident and
Emergency. The Protocol was designed to include
all patients with complaints of chest pain, excluding
those <12 years of age; in cardiac arrest; with
unmanageable airway or breathing; a Glasgow Coma
Scale score of ≤13; a first systolic blood pressure of
<90 mm Hg; a respiratory rate of <10 or >29 breaths
per minute; or refusal or inability to give consent.
Ambulances were equipped with 12-lead ECG
machines capable of automatic algorithm-based
diagnosis. The selected machine model was a corpuls3
Monitor and Defibrillator (GS Elektromedizinische
Geräte G Stemple GmbH, Kaufering, Germany),
with the telemedicine application corpuls.mission
(GS Elektromedizinische Geräte G Stemple GmbH,
Kaufering, Germany). The selected ECG algorithm
was the ECG diagnostic algorithm of the Hannover
ECG System (Corscience GmbH & Co KG, Erlangen,
Germany).
Upon encountering a patient who met the
Protocol’s criteria, the ambulance personnel
performed a 12-lead ECG on scene or in the
stationary ambulance compartment. The ECG was
immediately analysed by the computer algorithm
and classified as ‘STEMI’, ‘Not STEMI’, or ‘N/A’
(not interpretable due to suboptimal ECG quality).
Additional ECGs were performed as necessary to
improve quality. The ECG(s) were tele-transmitted
to the ED serving the particular catchment area
for reading and interpretation by the ED attending
physician. If the ECG was classified as ‘STEMI’ by
the computer algorithm, the EMS personnel also
directly called to alert the ED. The ED prepared the
resuscitation room for patient arrival if the ECG was
classified as STEMI by the ED physician.
Study population and data collection
This study adhered to the STARD (Standards
for Reporting of Diagnostic Accuracy Studies)
2015 reporting guideline. Patients with PHECGs
performed in accordance with the Protocol
throughout Hong Kong were prospectively recruited
from 1 October to 31 December 2021.
Prehospital ECGs performed and tele-transmitted
during the study period were obtained
from corpuls.mission’s online database and matched
to clinical data from the Clinical Data Analysis and
Reporting System and Accident and Emergency
Information System (Information Technology and
Health Informatics Division, Hospital Authority,
Hong Kong). Electrocardiograms without matching
patient data and those classified as ‘N/A’ by the
algorithm were excluded from the analysis.
Three reference standards were used to
investigate the diagnostic accuracy of the computer
algorithm. The first reference standard, the primary outcome, was adjudicated blinded rating of the ECG.
Each ECG was de-identified and independently
interpreted as ‘STEMI’, ‘Not STEMI’ or ‘Not
interpretable’ by two investigators: an emergency
physician with ≥5 years of experience in emergency
medicine practice and a specialist in Emergency
Medicine. Electrocardiograms for which there was
disagreement between the interpretations of the
two blinded raters were classified according to the
blinded interpretation of an adjudicator (a second
Emergency Medicine specialist). The diagnosis of
STEMI was based on the Fourth Universal Definition
of Myocardial Infarction14 and the modified Sgarbossa
criteria for left bundle branch block or ventricular
paced rhythm.15 16 ST-elevation myocardial infarction
mimics17 and STEMI equivalents, according to the
2022 ACC Expert Consensus Decision Pathway on
the Evaluation and Disposition of Acute Chest Pain
in the Emergency Department,18 were regarded as
‘Not STEMI’. ‘Not interpretable’ ECGs were those
with substantial motion artefacts, wavering baseline,
or disconnected lead(s); these ECGs were excluded
from the analysis.
The second reference standard was the ED
attending physician’s diagnosis, which considered
the patient’s clinical condition, along with additional
ECGs and other investigations performed upon
arrival in the ED. Patients without ECGs performed
in the ED were excluded from the analysis.
The third reference standard was the diagnosis
on hospital discharge from the index admission. We
excluded patients who died in the ED without an
established diagnosis, who developed STEMI after
admission, or were discharged with acknowledgement
of medical advice and no definitive diagnosis.
Interrater agreement analysis was performed in
three dimensions, namely, between the two blinded
raters, between the adjudicated blinded rating and
the ED diagnosis, and between the adjudicated
blinded rating and the diagnosis on hospital
discharge. If there was disagreement between the
adjudicated blinded rating and the ED diagnosis,
the prehospital and ED ECGs were reviewed by
the principal investigator to differentiate between
dynamic change or true disagreement. Dynamic
change was defined as the lack of ST-segment
elevation and ECG criteria fulfilment on the initial
PHECG, with subsequent evidence on serial ECG
performed in the ED.
False-positive and false-negative ECGs were
reviewed and classified by the principal investigator.
The following categories of ECG morphology were
determined based on criteria described in existing
literature: Brugada pattern,19 early repolarisation,20
left bundle branch block or paced rhythm not
matching STEMI criteria,15 16 left ventricular
hypertrophy,21 pericarditis,22 and ventricular
ectopics.23
Statistical analysis
Continuous variables were presented as mean ± standard deviation and were analysed with the
independent t test. Categorical variables were
reported as absolute frequencies and percentages
and were analysed with the Chi squared test or
Fisher’s exact test. Interrater agreement regarding
ECG diagnosis was analysed using Cohen’s kappa.
Sensitivity, specificity, and predictive values were
derived from 2 × 2 contingency tables and analysed
with the Chi squared test.
The threshold for statistical significance was
regarded as P<0.05. All statistical analyses were
performed using SPSS software (Windows version
26.0; IBM Corp, Armonk [NY], US).
Results
Baseline characteristics
During the study period, 2801 PHECGs were performed, one for each patient who presented with
chest pain. Of these ECGs, 2437 were matched to
electronic patient records. After the exclusion of 103
ECGs classified as ‘N/A’ by the computer algorithm,
2334 ECGs were included in the analysis (Fig 1).
The characteristics of the study population are
presented in Table 1. Overall, 62.9% of the patients
were men. The mean age of male patients, female
patients, and both sexes were 63.9 years, 74.1 years,
and 67.7 years, respectively. In total, 83.6% of patients
were placed on stretchers upon arrival at the ED.
Furthermore, 8.2% of patients were institutionalised
in residential homes. Of the ECGs, 42.4% were
performed during 0800 to 1559 hours, 35.4% were
performed during 1600 to 2359 hours, and 22.3%
were performed during 0000 to 0759 hours. A total
of 405 (17.4%) PHECGs were classified as STEMI by
the algorithm.
Primary outcome
The primary outcome was diagnostic accuracy based
on the adjudicated blinded rating. The prevalence
of STEMI was 5.1% (Table 2). There was good
interrater observed agreement (96.9%) between
the two blinded ECG assessors. Cohen’s kappa
was 0.84 (95% confidence interval [CI]=0.81-0.88;
P<0.05) [Table 3]. The algorithm had a sensitivity of
94.6% (95% CI=88.2%-97.8%), specificity of 87.9%
(95% CI=86.4%-89.2%), positive predictive value of
29.4% (95% CI=24.8%-34.4%), negative predictive
value of 99.7% (95% CI=99.3%-99.9%), positive
likelihood ratio of 7.8 (95% CI=6.9-8.8), and negative
likelihood ratio of 0.06 (95% CI=0.03-0.13) [all
P<0.05] (Table 2).
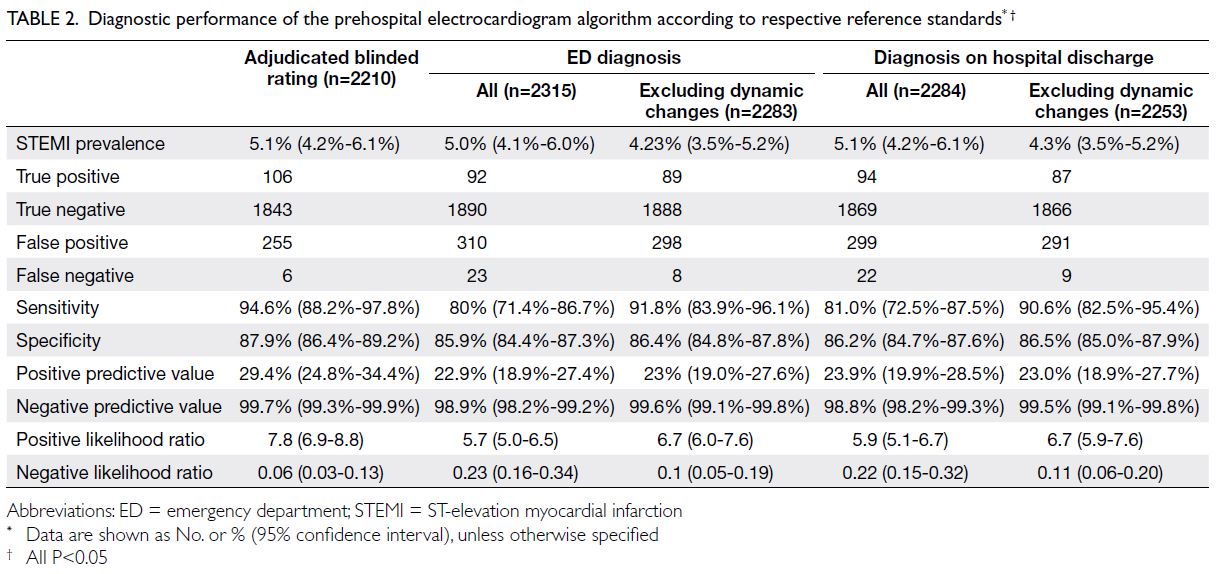
Table 2. Diagnostic performance of the prehospital electrocardiogram algorithm according to respective reference standards
Secondary outcomes
Secondary outcomes were the algorithm’s diagnostic accuracy with reference to the ED attending
physician’s diagnosis and to the diagnosis on hospital
discharge.
Substantial agreement was observed between
the diagnosis based on the adjudicated blinded rating
and these two reference standards. Discrepancies in
agreement between the adjudicated blinded rating
of ECGs and these two reference standards reflected
the presence of dynamic ECG changes. Observed
agreement between the adjudicated blinded rating
and ED physician’s diagnosis was 97.1%, with Cohen’s
kappa of 0.69. Excluding patients with dynamic
ECG changes in the ED, the observed agreement
was 98.2% and Cohen’s kappa was 0.78. Observed
agreement between the adjudicated blinded rating
and final discharge diagnosis was 97.5%, with Cohen’s
kappa of 0.74. Excluding patients with dynamic ECG
changes in the ED, the observed agreement was 98.4% and Cohen’s kappa was 0.80 (Table 3). The
diagnostic performance based on the three reference
standards and the analysis of interrater agreement
are summarised in Tables 2 and 3, respectively.
Characteristics of false-positive electrocardiograms
The 255 false-positive ECGs with the adjudicated
blinded rating as the reference standard were
reviewed and characterised as shown in Figure 2.
The leading causes were early repolarisation (n=97;
38.0%), left bundle branch block (n=40; 15.7%), and
tachycardia of >140 beats per minute (n=34; 13.3%).
Excluding ECGs with suboptimal quality (classified
as ‘N/A’ by the algorithm and ‘Not interpretable’
according to adjudicated blinded rating), false-positive
ECGs due to artefacts constituted 8.6% (n=22).
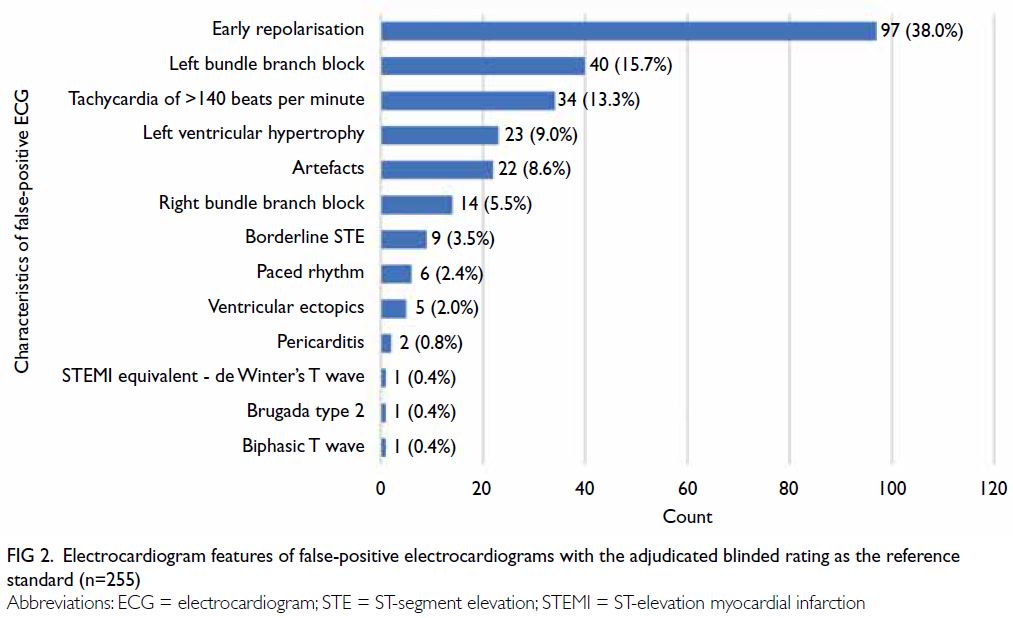
Figure 2. Electrocardiogram features of false-positive electrocardiograms with the adjudicated blinded rating as the reference standard (n=255)
Characteristics of false-negative
electrocardiograms
Using the diagnosis on hospital discharge as the
reference standard, 22 STEMI cases were missed by the algorithm (Fig 3). Thirteen (59.1%) of the
false-negative ECGs were due to the development
of dynamic ECD changes in the ED; four (18.2%) of
these had subtle ST-segment elevation. ST-segment elevation in lead augmented vector right and the
STEMI equivalent morphology of de Winter’s T
wave were noted in two (9.1%) ECGs each. One ECG
was classified as ‘Not interpretable’ according to the
adjudicated blinded rating because of substantial
artefacts.
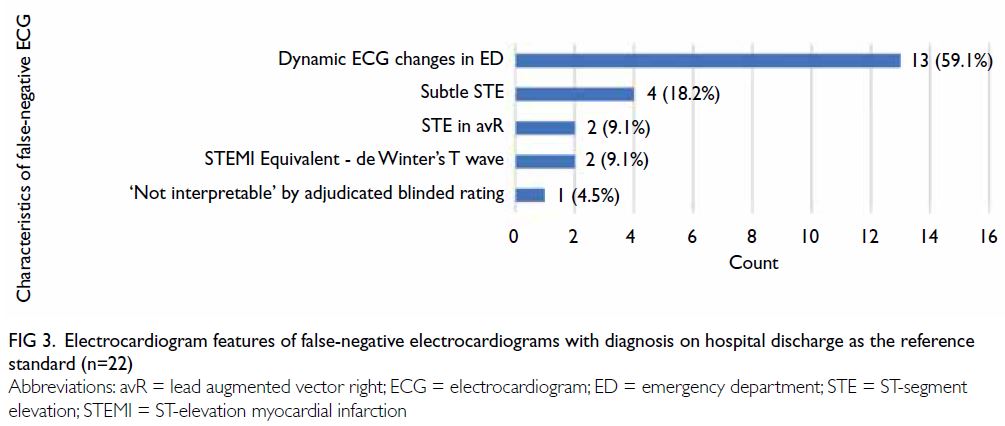
Figure 3. Electrocardiogram features of false-negative electrocardiograms with diagnosis on hospital discharge as the reference standard (n=22)
Discussion
Implications on prehospital care systems for
ST-elevation myocardial infarction
This prospective observational study examined the
diagnostic performance of a rule-based PHECG
algorithm universally utilised in Hong Kong, based
on three levels of reference standards. The primary
outcome, adjudicated blinded rating, closely reflects
diagnostic performance without the addition of patient clinical history and presentation or any other
diagnostic aids. The American Heart Association
recommends three levels of PHECG diagnosis,
namely, EMS interpretation, computerised algorithm
diagnosis, and ECG transmission for remote
interpretation.24 However, in healthcare systems
such as the Hospital Authority in Hong Kong, EMS
are trained to perform but not interpret PHECGs.
Thus, it is important to understand reliance on the
computerised algorithm using the benchmark of
physician-based remote interpretation; these data
can guide the establishment and improvement of
care systems.
One in eight of the PHECGs in this study
showed false-positive results. Considering the
fair specificity and positive predictive value of
only 29.4% for the automated ECG diagnostic programme, this high false-positive rate reflected
limitations in guiding prehospital treatment and
streamlining care systems (eg, prehospital diversion
to percutaneous coronary intervention–capable
centres or prehospital triage for direct transfer
to a cardiac catheterisation laboratory). A hybrid
two-step ECG interpretation model, involving
a physician’s remote (ie, telemedicine-based)
interpretation of ECGs that are classified as STEMI
by the computerised algorithm, could be adopted
to minimise overactivation and ensure prudent use
of healthcare resources. Nonetheless, the algorithm
exhibited good sensitivity in terms of identifying
STEMI patients. Its high negative predictive
value allowed STEMI to be reasonably excluded
based on ECG results. Although remote PHECG
interpretation is considered relatively accurate, it
generally results in STEMI misdiagnosis rates of 6%
to 8%.13 Therefore, we included secondary outcomes,
namely, the algorithm’s diagnostic performance
based on the ED attending physician’s diagnosis and
the final discharge diagnosis; we assessed interrater
agreement between these reference standards. We
adopted an operational approach focused on the
‘appropriateness of cardiac catheterisation laboratory
activation’, rather than a strictly patient-centred
approach based on primary percutaneous coronary
intervention findings or cardiac biomarkers.
Diagnostic performance varies across
electrocardiogram machine models and algorithms
The inclusion of three reference standards was
intended to address the heterogeneous estimates
of PHECG diagnostic performance for STEMI
in existing literature. Prior studies have been
based on various reference standards, including blinded physician rating,25 ED attending physician’s
diagnosis,6 26 hospital discharge diagnosis,7 and
the appropriateness of coronary angiography
activation.8 The results have varied according to
STEMI prevalence in the study population, as
well as the reference standard, ECG machine, and
computerised algorithm. Using ED clinical diagnosis
as the reference standard, a single-centre pilot study
in Hong Kong by Cheung et al6 utilising the X Series
Monitor/Defibrillator and Inovise 12L Interpretive
Algorithm (Zoll Medical Corporation, Chelmsford
[MA], US) demonstrated a low sensitivity (53.8%)
and high specificity (99.6%). Bhalla et al26 utilised
LIFEPAK 12 monitors (Physio-Control, Redmond
[WA], US) equipped with a Marquette 12SL ECG
analysis programme (General Electric Company,
Fairfield [CT], US) to evaluate PHECGs from 100
STEMI patients and 100 control participants; they
found a similarly low sensitivity (58%) and very
high specificity (100%). Bosson et al7 examined
ECGs obtained with the LIFEPAK 15 monitor
(Physio-Control, Inc, Minneapolis [MN], US) and
analysed using the University of Glasgow 12-Lead
ECG Analysis Programme (version 27); their results
showed 92.8% sensitivity and 98.7% specificity, based
on the reference standard of appropriateness for
emergency coronary angiography.7 The prevalence
of STEMI was much lower in their study than in our
study (1.4%7 vs 5.1% [Table 2]) because their dataset
also included PHECGs performed for symptoms
other than chest pain. Using the same ECG machine
model as the aforementioned study,7 Fakhri et al8
tested an automated analysis method with a high-specificity
STEMI configuration. In a carefully
selected STEMI population, the sensitivity and
specificity were 69.8% and 51.5%, respectively, based
on discharge diagnosis.8 A meta-analysis conducted by Tanaka et al27 suggested that computer-assisted
ECG interpretation had a high pooled specificity
(95.4%; 95% CI=87.3%-98.4%) with an acceptable
estimated number of false-positive results, whereas
the pooled sensitivity was relatively low (85.4%;
95% CI=74.1%-92.3%), for identifying STEMI on
PHECG. All of these studies utilised ECG machines
and diagnostic algorithms that differed from our
method, emphasising that diagnostic performance
varies across models; evaluations of specific ECG
machines and algorithms should be conducted
by individual healthcare systems to suit their
operational needs.
Major patterns of false-positive and falsenegative
electrocardiograms
The Hannover ECG System algorithm utilised in
our study was one of nine computer programmes
investigated in the international Common Standards
for Quantitative Electrocardiography Diagnostic
Study,28 using clinical diagnosis as the reference
standard. This statistics-based algorithm exhibited
one of the highest sensitivities (79.0%) for detecting
myocardial infarction compared with all algorithms
combined (72.2%); its sensitivity also was similar to
that of the combined independent ratings of eight
cardiologists (80.3%). However, its ability to correctly
classify normal ECGs (86.6%) was lower than that of
the combined ratings of cardiologists (97.1%) and
the combined algorithms (96.7%). Our findings are
consistent with the results of the Common Standards
for Quantitative Electrocardiography Diagnostic
Study. The presence of artefacts contributed to 8.6%
of false-positive ECGs; this rate could be improved
by enhancing ECG technique. The major patterns
of misdiagnosis were early repolarisation (38.0%),
left bundle branch block (15.7%), and tachycardia
of >140 beats per minute (13.3%) [Fig 2]. Artefacts
on ECG were responsible for the largest proportion
of false-positive ECGs8; they contributed a smaller
proportion in our dataset because we excluded ECGs
considered ‘Not interpretable’ by the algorithm
or blinded raters. Early repolarisation remained a
leading cause of false-positive ECGs, and existing
consensus papers on early repolarisation may help
guide future algorithm development.20 29 Further
collaboration with the software provider to optimise
the algorithm may enhance its accuracy.
Among cases of STEMI missed by the
algorithm using diagnosis on hospital discharge
as the reference standard, more than half were
caused by ECG changes after patient arrival in the
ED. False-negative ECGs due to subtle ST-segment
elevation represented only 3.45% of all STEMI
patients. Remote physician interpretation of these
PHECGs would likely be equivocal and uncertain.
It presumably would not be beneficial to adjust the
algorithm to correct this margin of error, considering the potential for additional false-positives. However,
it might be useful to refine the algorithm for
enhanced detection of STEMI equivalents, which
were missed in the current cohort.
The rise of artificial intelligence
Although the diagnostic limitations of rule-based
algorithms are recognised, Zhao et al9 described
an artificial intelligence diagnostic algorithm that
showed promising results (96.8% sensitivity and 99%
specificity) using coronary angiography findings as
the reference standard. The potential role of artificial
intelligence in PHECG diagnosis merits further
exploration to increase accuracy.
Paradigm shift in classifying myocardial
infarction
Meyers et al30 proposed a new paradigm of occlusion
myocardial infarction (OMI) vs non-OMI, which
they compared with the conventional STEMI vs
non-STEMI paradigm. Occlusion myocardial
infarction refers to type 1 myocardial infarction that
involves acute total or near-total occlusion of a major
epicardial coronary vessel with insufficient collateral
circulation, causing acute infarction. Meyers et al30
showed that 38% of OMI patients did not meet ECG-based
STEMI criteria, as stated in the 4th Universal
Definition of Myocardial Infarction.14 Compared
with OMI patients who met STEMI criteria, patients
not meeting the criteria experienced significant
delays in cardiac catheterisation but exhibited
similar adverse outcome profiles. These findings
highlight the need to re-evaluate classification
strategies for acute coronary syndrome, with a focus
on rapidly recognising this underserved and poorly
understood subgroup of patients who would benefit
from emergent reperfusion therapy. Future research
should emphasise identifying ECG features of OMI
beyond the STEMI criteria.
Limitations
First, 13% of PHECGs were not matched to
electronic patient records, resulting in the loss of
data for interpretation. Second, during adjudicated
blinded rating of the ECGs, STEMI equivalents
were not included in the definition of STEMI
because the algorithm was not designed to include
these characteristics. This exclusion differs from
real-world scenarios in which the recognition of
STEMI equivalents would prompt ED physicians to
implement STEMI management. Third, this study
evaluated a single rule-based algorithm combined
with a single ECG machine model utilised by a single
urban EMS service provider serving a predominantly
ethnic Chinese population. Fourth, intraobserver
variability was not assessed for each ECG reviewer.
Finally, ECGs considered ‘Not interpretable’ by ECG reviewers due to substantial artefacts were
excluded from data analysis, which might lead to
underestimation regarding the contributions of
artefacts to false positivity.
Conclusion
In this territory-wide study, a rule-based PHECG algorithm demonstrated good sensitivity and fair
specificity for the diagnosis of STEMI.
Author contributions
Concept or design: JHY Lai, CT Lui.
Acquisition of data: JHY Lai, TWT Chan, BCP Wong.
Analysis or interpretation of data: JHY Lai, CT Lui.
Drafting of the manuscript: JHY Lai.
Critical revision of the manuscript for important intellectual content: CT Lui, TWT Chan, BCP Wong, MSH Tsui, BKA Wan, KL Mok.
Acquisition of data: JHY Lai, TWT Chan, BCP Wong.
Analysis or interpretation of data: JHY Lai, CT Lui.
Drafting of the manuscript: JHY Lai.
Critical revision of the manuscript for important intellectual content: CT Lui, TWT Chan, BCP Wong, MSH Tsui, BKA Wan, KL Mok.
All authors had full access to the data, contributed to the study, approved the final version for publication, and take responsibility for its accuracy and integrity.
Conflicts of interest
All authors have disclosed no conflicts of interest.
Funding/support
This research received no specific grant from any funding agency in the public, commercial, or not-for-profit sectors.
Ethics approval
The research was approved by the New Territories West Cluster Research Ethics Committee of Hospital Authority, Hong Kong (Ref No.: NTWC/REC/21097). The requirement
for patient consent was waived by the Committee because the
study was conducted within a preexisting prehospital clinical
service.
References
1. HealthyHK, Hong Kong SAR Government. Coronary heart
diseases. 2021. Available from: https://www.healthyhk.gov.hk/phisweb/en/chart_detail/24/. Accessed 3 Dec 2022.
2. O’Gara PT, Kushner FG, Ascheim DD, et al. 2013 ACCF/AHA guideline for the management of ST-elevation
myocardial infarction: executive summary: a report of the
American College of Cardiology Foundation/American
Heart Association Task Force on Practice Guidelines. J Am
Coll Cardiol 2013;61:485-510. Crossref
3. Ibánez B, James S, Agewall S, et al. 2017 ESC Guidelines
for the management of acute myocardial infarction in
patients presenting with ST-segment elevation [in English,
Spanish]. Rev Esp Cardiol (Engl Ed) 2017;70:1082. Crossref
4. Kontos MC, Gunderson MR, Zegre-Hemsey JK, et al.
Prehospital activation of hospital resources (PreAct)
ST-segment-elevation myocardial infarction (STEMI):
a standardized approach to prehospital activation
and direct to the catheterization laboratory for
STEMI recommendations from the American Heart
Association’s mission: lifeline program. J Am Heart Assoc 2020;9:e011963. Crossref
5. Brunetti ND, De Gennaro L, Correale M, et al. Prehospital
electrocardiogram triage with telemedicine near
halves time to treatment in STEMI: a meta-analysis and
meta-regression analysis of non-randomized studies. Int J
Cardiol 2017;232:5-11. Crossref
6. Cheung KS, Leung LP, Siu YC, et al. Prehospital 12-lead
electrocardiogram for patients with chest pain: a pilot
study. Hong Kong Med J 2018;24:484-91. Crossref
7. Bosson N, Sanko S, Stickney RE, et al. Causes of prehospital
misinterpretations of ST elevation myocardial infarction.
Prehosp Emerg Care 2017;21:283-90. Crossref
8. Fakhri Y, Andersson H, Gregg RE, et al. Diagnostic
performance of a new ECG algorithm for reducing false
positive cases in patients suspected acute coronary
syndrome. J Electrocardiol 2021;69:60-4. Crossref
9. Zhao Y, Xiong J, Hou Y, et al. Early detection of ST-segment
elevated myocardial infarction by artificial intelligence with
12-lead electrocardiogram. Int J Cardiol 2020;317:223-30. Crossref
10. Goebel M, Vaida F, Kahn C, Donofrio JJ. A novel algorithm
for improving the diagnostic accuracy of prehospital STelevation
myocardial infarction. Prehosp Disaster Med
2019;34:489-96. Crossref
11. Le May MR, Dionne R, Maloney J, et al. Diagnostic
performance and potential clinical impact of advanced
care paramedic interpretation of ST-segment elevation
myocardial infarction in the field. CJEM 2006;8:401-7. Crossref
12. Ducas RA, Wassef AW, Jassal DS, et al. To transmit or not
to transmit: how good are emergency medical personnel in
detecting STEMI in patients with chest pain? Can J Cardiol
2012;28:432-7. Crossref
13. Tanguay A, Lebon J, Brassard E, Hébert D, Bégin F.
Diagnostic accuracy of prehospital electrocardiograms
interpreted remotely by emergency physicians in
myocardial infarction patients. Am J Emerg Med
2019;37:1242-7. Crossref
14. Thygesen K, Alpert JS, Jaffe AS, et al. Fourth universal
definition of myocardial infarction (2018). J Am Coll
Cardiol 2018;72:2231-64. Crossref
15. Meyers HP, Limkakeng AT Jr, Jaffa EJ, et al. Validation of the
modified Sgarbossa criteria for acute coronary occlusion
in the setting of left bundle branch block: a retrospective
case-control study. Am Heart J 2015;170:1255-64. Crossref
16. Smith SW, Dodd KW, Henry TD, Dvorak DM, Pearce LA.
Diagnosis of ST-elevation myocardial infarction in the
presence of left bundle branch block with the ST-elevation
to S-wave ratio in a modified Sgarbossa rule. Ann Emerg
Med 2012;60:766-76. Crossref
17. Wang K, Asinger RW, Marriott HJ. ST-segment elevation
in conditions other than acute myocardial infarction. N
Engl J Med 2003;349:2128-35. Crossref
18. Writing Committee; Kontos MC, de Lemos JA, et al.
2022 ACC Expert Consensus Decision Pathway on the
evaluation and disposition of acute chest pain in the
emergency department: a report of the American College
of Cardiology Solution Set Oversight Committee. J Am
Coll Cardiol 2022;80:1925-60. Crossref
19. Wilde AA, Antzelevitch C, Borggrefe M, et al. Proposed
diagnostic criteria for the Brugada syndrome: consensus
report. Circulation 2002;106:2514-9. Crossref
20. Patton KK, Ellinor PT, Ezekowitz M, et al.
Electrocardiographic early repolarization: a scientific
statement from the American Heart Association. Circulation 2016;133:1520-9. Crossref
21. Armstrong EJ, Kulkarni AR, Bhave PD, et al.
Electrocardiographic criteria for ST-elevation myocardial
infarction in patients with left ventricular hypertrophy. Am
J Cardiol 2012;110:977-83. Crossref
22. Bischof JE, Worrall C, Thompson P, Marti D, Smith SW. ST
depression in lead aVL differentiates inferior ST-elevation
myocardial infarction from pericarditis. Am J Emerg Med
2016;34:149-54. Crossref
23. Mond HG, Haqqani HM. The electrocardiographic
footprints of ventricular ectopy. Heart Lung Circ
2020;29:988-99. Crossref
24. Ting HH, Krumholz HM, Bradley EH, et al. Implementation
and integration of prehospital ECGs into systems of
care for acute coronary syndrome: a scientific statement
from the American Heart Association Interdisciplinary
Council on Quality of Care and Outcomes Research,
Emergency Cardiovascular Care Committee, Council
on Cardiovascular Nursing, and Council on Clinical
Cardiology. Circulation 2008;118:1066-79. Crossref
25. Wilson RE, Kado HS, Percy RF, et al. An algorithm for identification of ST-elevation myocardial infarction
patients by emergency medicine services. Am J Emerg Med
2013;31:1098-102. Crossref
26. Bhalla MC, Mencl F, Gist MA, Wilber S, Zalewski J.
Prehospital electrocardiographic computer identification
of ST-segment elevation myocardial infarction. Prehosp
Emerg Care 2013;17:211-6. Crossref
27. Tanaka A, Matsuo K, Kikuchi M, et al. Systematic review
and meta-analysis of diagnostic accuracy to identify ST-segment
elevation myocardial infarction on interpretations
of prehospital electrocardiograms. Circ Rep 2022;4:289-97. Crossref
28. Willems JL, Abreu-Lima C, Arnaud P, et al. The diagnostic
performance of computer programs for the interpretation
of electrocardiograms. N Engl J Med 1991;325:1767-73. Crossref
29. Macfarlane PW, Antzelevitch C, Haissaguerre M, et al. The
early repolarization pattern: a consensus paper. J Am Coll
Cardiol 2015;66:470-7. Crossref
30. Meyers HP, Bracey A, Lee D, et al. Comparison of the ST-elevation
myocardial infarction (STEMI) vs. NSTEMI and
occlusion MI (OMI) vs. NOMI paradigms of acute MI. J
Emerg Med 2021;60:273-84. Crossref