Hong Kong Med J 2024 Dec;30(6):468–77 | Epub 19 Dec 2024
© Hong Kong Academy of Medicine. CC BY-NC-ND 4.0
ORIGINAL ARTICLE
Artificial intelligence–based computer-aided diagnosis for breast cancer detection on digital mammography in Hong Kong
SM Yu, MB, BS, FHKAM (Radiology)1; Catherine YM Young, MB, BS, FRCR1; YH Chan, MB, ChB, FRCR1; YS Chan, MB, ChB, FHKAM (Radiology)1; Carita Tsoi, MB, ChB, FHKAM (Radiology)1; Melinda NY Choi, MHSc, GCB1; TH Chan, BSc, MSc1; Jason Leung, MSc2; Winnie CW Chu, MB, ChB, FHKAM (Radiology)1; Esther HY Hung, MB, ChB, FHKAM (Radiology)1; Helen HL Chau, MB, ChB, FHKAM (Radiology)
1 Department of Imaging and Interventional Radiology, Prince of Wales Hospital, Hong Kong SAR, China
2 The Jockey Club Centre for Osteoporosis Care and Control, The Chinese University of Hong Kong, Hong Kong SAR, China
Corresponding author: Dr SM Yu (ysm687@ha.org.hk)

Abstract
Introduction: Research concerning artificial
intelligence in breast cancer detection has primarily
focused on population screening. However, Hong
Kong lacks a population-based screening programme.
This study aimed to evaluate the potential of artificial
intelligence–based computer-assisted diagnosis (AI-CAD)
program in symptomatic clinics in Hong Kong
and analyse the impact of radio-pathological breast
cancer phenotype on AI-CAD performance.
Methods: In total, 398 consecutive patients with
414 breast cancers were retrospectively identified
from a local, prospectively maintained database
managed by two tertiary referral centres between
January 2020 and September 2022. The full-field
digital mammography images were processed using
a commercial AI-CAD algorithm. An abnormality
score <30 was considered a false negative, whereas
a score of ≥90 indicated a high-score tumour.
Abnormality scores were analysed with respect to
the clinical and radio-pathological characteristics of
breast cancer, tumour-to–breast area ratio (TBAR),
and tumour distance from the chest wall for cancers
presenting as a mass.
Results: The median abnormality score across the
414 breast cancers was 95.6; sensitivity was 91.5%
and specificity was 96.3%. High-score cancers were
more often palpable, invasive, and presented as
masses or architectural distortion (P<0.001). False-negative
cancers were smaller, more common in dense breast tissue, and presented as asymmetrical densities (P<0.001). Large tumours with extreme
TBARs and locations near the chest wall were
associated with lower abnormality scores (P<0.001).
Several strengths and limitations of AI-CAD were
observed and discussed in detail.
Conclusion: Artificial intelligence–based computer-assisted
diagnosis shows potential value as a tool
for breast cancer detection in symptomatic setting,
which could provide substantial benefits to patients.
New knowledge added by this study
- With a threshold score of 30, a commercially available artificial intelligence–based computer-assisted diagnosis (AI-CAD) program showed high sensitivity and specificity for breast cancer detection on digital mammography in symptomatic settings, offering a valuable diagnostic adjunct.
- The performance of AI-CAD varied according to the radio-pathological characteristics of breast cancer. Notably, the program demonstrated promising accuracy in detecting breast cancers that exhibit architectural distortion, which remains a diagnostic challenge.
- Observed limitations of AI-CAD, such as underscoring cancers that present as large masses or exhibit nipple retraction as well as its inability to compare with previous studies, highlight concerns regarding standalone use of AI for triage in symptomatic clinics.
- Artificial intelligence–based computer-assisted diagnosis exhibits substantial potential for detecting breast cancers in symptomatic settings.
- To make study findings clinically viable, larger validation studies are needed.
Introduction
Mammography is the principal modality used for
breast cancer screening and detection in women
worldwide.1 However, 10% to 30% of breast
cancers may be undetected during mammography
due to factors such as dense breast tissue, poor
imaging technique, perceptual error, and subtle
mammographic abnormalities.2
Conventional computer-aided diagnosis
systems have been developed for more than two
decades; however, large-scale studies have shown
no significant benefit of such systems in enhancing
radiologists’ diagnostic performance.3 4 Such systems
do not facilitate differentiation between benign and
malignant breast lesions, resulting in numerous
false-positive results that require radiologist review,
which may lead to reader fatigue and unnecessary
additional investigations.
Currently, artificial intelligence–based
computer-assisted diagnosis (AI-CAD) is widely
implemented in mammography to improve
diagnostic accuracy and reduce radiologist
workload.5 6 The AI-CAD systems developed using
deep-learning algorithms make independent
decisions and self-learn without the need for
feature engineering and computation.7 Artificial
intelligence algorithms have been applied to multiple
aspects of breast cancer screening, including risk
stratification, triage, lesion interpretation, and patient recall.8 As of 2022, the US Food and
Drug Administration has approved >15 AI
tools for mammography applications, including
density assessment, triage, lesion detection, and
classification.9 Most commercial AI-CAD programs
provide heatmaps with abnormality scores.
Generally, higher abnormality scores indicate
more suspicious radiological features and a greater
likelihood of cancer.
Most existing evidence in the literature is
derived from population-based screening studies.5 10 11
However, unlike other developed Asian countries
such as Singapore and Korea, Hong Kong lacks a
large-scale population screening programme.12 13
Our patient population primarily consists of
symptomatic individuals. Evidence concerning
the application of AI-CAD in symptomatic breast
imaging is limited. This study aimed to evaluate
the potential of AI-CAD in Hong Kong, focusing
on the impact of radio-pathological phenotypes of
breast cancer on AI-CAD performance. We analysed
the distinctive characteristics of high-score versus
low-score breast cancers. We also discuss observed
strengths and limitations of AI-CAD in identifying
breast cancer.
Methods
Study population
In total, 488 consecutive patients with histology-confirmed
breast cancers were identified from a
prospectively maintained database managed by two
tertiary referral centres in Hong Kong during the
period between January 2020 and September 2022.
In our centres, all patients referred for diagnostic
mammography were symptomatic, presenting with
various clinical symptoms. We included patients
with breast cancers confirmed by core needle
biopsy under ultrasound guidance or stereotactic-guided
vacuum-assisted breast biopsy performed at
our centres. We excluded patients with diagnostic
mammography performed at outside facilities (n=6),
chest wall recurrence after mastectomy (n=14),
cancers identified only in axillary nodes (n=3),
tumour locations not feasible for mammography
(n=3), and mammographically occult breast cancers
undetectable by both reporting radiologists and
AI-CAD (n=64) [Fig 1]. Finally, 398 patients with
414 breast cancers and 347 unaffected breasts
were included in the study. Sixteen patients were
diagnosed with bilateral breast cancer. Among the
382 patients with unilateral breast cancer, 35 had
previously undergone contralateral mastectomy.
Image acquisition and analysis
Full-field digital mammography (MAMMOMAT Inspiration; Siemens, Erlangen, Germany or
Selenia Dimensions; Hologic, Newark [DE], US) was performed prior to each biopsy. The included mammograms were exported and processed by a
commercial AI-CAD program (INSIGHT MMG,
version 1.10.2; Lunit, Seoul, South Korea), which is
approved by the US Food and Drug Administration
for lesion detection and classification in breast imaging.9
The AI-CAD algorithm used in the current study
was developed and validated through multinational
studies.14 15 This algorithm provides a heatmap
that highlights mammographic abnormalities and
generates a score ranging from 0 to 100 for each view
(craniocaudal and mediolateral oblique views). The
abnormality score is the maximum value for each
breast, reflecting the likelihood of malignancy.
All mammograms were interpreted by
radiologists subspecialising in breast radiology
(with 4 to 20 years of experience in breast imaging).
Mammography reports from the time of breast
cancer diagnosis were retrieved from the radiology
information system and retrospectively reviewed for
breast density, dominant mammographic features of
breast cancer, and any axillary lymphadenopathy. The
clinical findings, pathological results, and molecular
profiles of breast cancers were also recorded.
Breast density was categorised from 1 to 4 using
the BI-RADS (Breast Imaging Reporting and Data
System) classification.16 The cancers were classified
according to their dominant mammographic features
as asymmetrical density, mass (with or without
calcifications), calcifications alone, or architectural
distortion.
For breast cancers presenting as a mass
without calcifications, the tumour distances from
the chest wall and the tumour-to–breast area ratio
(TBAR) were measured in mammograms using the
picture archiving and communication system by
a radiologist with 2 years of experience in breast
imaging. Tumour distance from the chest wall was defined as the shortest distance between the tumour
and the pectoralis major in the mediolateral oblique
view (Fig 2a). Tumours partially visible within
the lower breast in the mediolateral oblique view,
where the pectoralis muscle is not discernible, were
assigned a chest wall distance of 0 cm. The TBAR
was calculated via division of the tumour area by the
breast area, as measured using the freehand region-of-interest tool (Fig 2b).
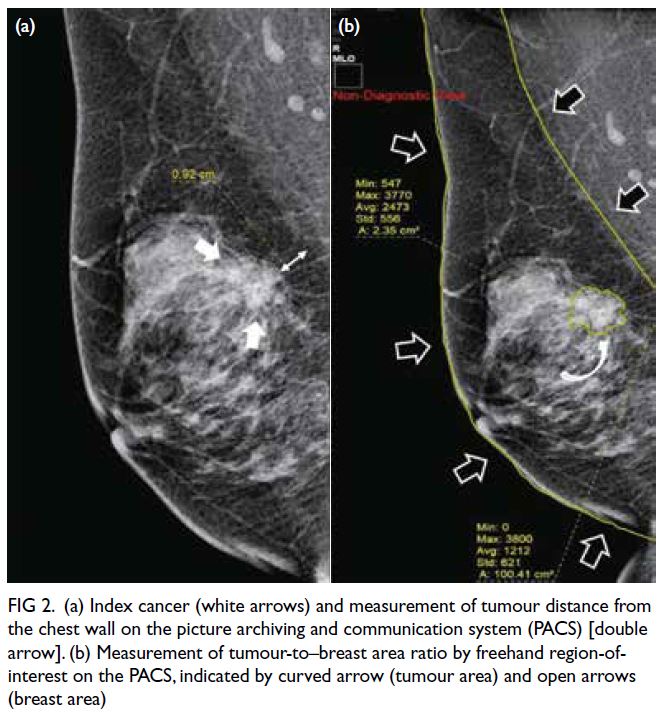
Figure 2. (a) Index cancer (white arrows) and measurement of tumour distance from the chest wall on the picture archiving and communication system (PACS) [double arrow]. (b) Measurement of tumour-to–breast area ratio by freehand region-of-interest on the PACS, indicated by curved arrow (tumour area) and open arrows (breast area)
The radiologists matched the index lesion to
the AI-CAD heatmap to determine whether the
AI-CAD correctly localised the known cancer. When
the cancer was correctly localised by the AI-CAD,
an abnormality score of ≥30 was regarded as a true positive, whereas a score <30 was considered a
false negative. When the cancer was undetected or
incorrectly localised by the AI-CAD, this result also
was regarded as a false negative. Breast cancers with
abnormality scores of ≥90 and <30 were designated
as ‘high-score tumour’ and ‘low-score tumour’, respectively.
Statistical analysis
Abnormality scores are presented as medians with
interquartile ranges. The scores were analysed
according to patient symptoms, breast density,
mammographic findings, cancer histology, and
molecular profile using the Mann-Whitney U test
or Kruskal–Wallis H test. The AI-CAD abnormality
scores were divided into three intervals: 0 to <30,
30-90, and >90 to 100. The Chi squared test and
Mantel-Haenszel test for trend were used to analyse
associations with different factors. For cancers
presenting as a mass, mean abnormality scores
across various TBARs and distances to the chest
wall were evaluated using analysis of variance with
pairwise comparisons. Statistical analyses were
performed using SPSS (Windows version 26; IBM
Corp, Armonk [NY], US). P values <0.05 were
considered statistically significant.
Results
In total, 398 patients (mean age, 62.4 years; range,
35-100) with 414 breast cancers and 347 unaffected
breasts were included in the study. The cohort
consisted of two men and 396 women. Among the
414 breast cancer cases, 284 (68.6%) were palpable
(Table 1).
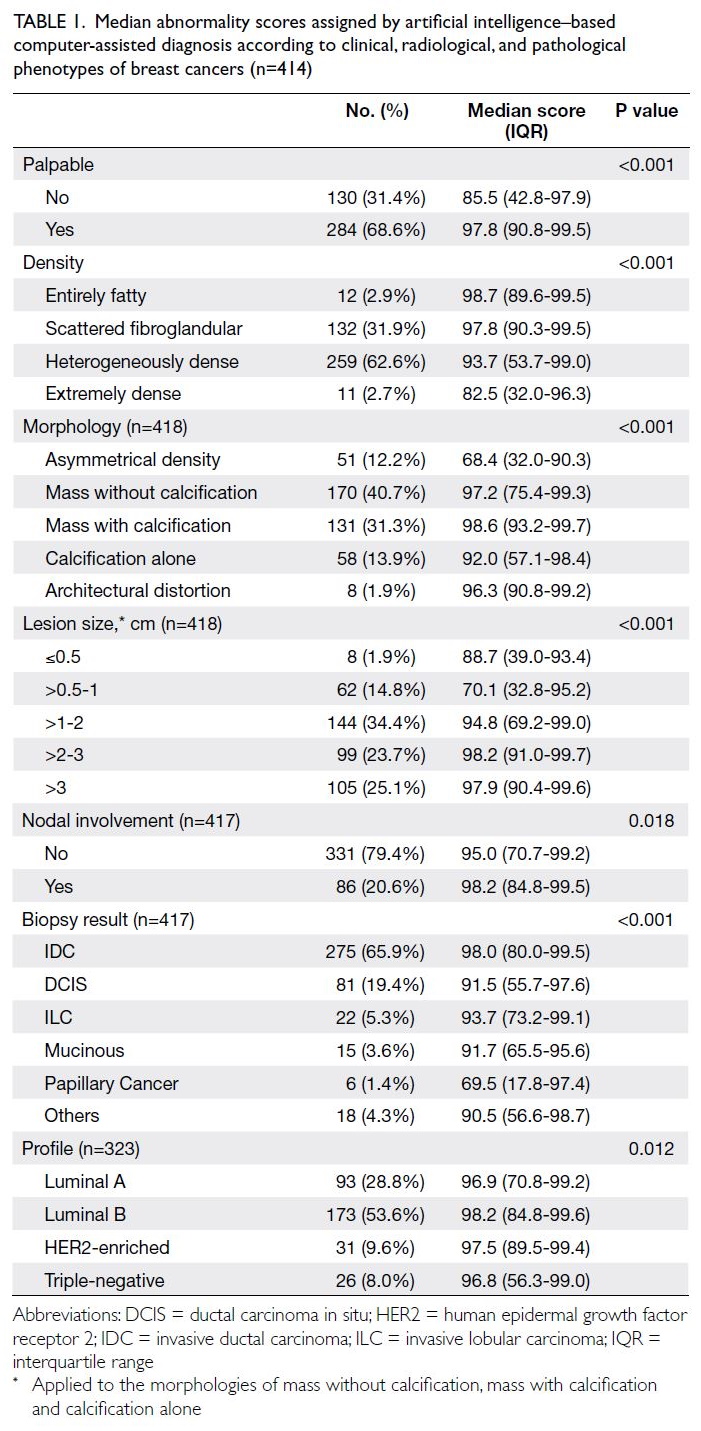
Table 1. Median abnormality scores assigned by artificial intelligence–based computer-assisted diagnosis according to clinical, radiological, and pathological phenotypes of breast cancers (n=414)
Distribution of abnormality scores
The median and mean abnormality scores for the
414 breast cancers were 95.6 and 80.6, respectively
(range, 0.4-99.9). The distribution of breast cancers
according to abnormality score interval is presented
in Figure 3. The sensitivity of the AI-CAD algorithm
in detecting breast cancers was 91.5%, based on
breast cancer identification using an abnormality
score of ≥30. Overall, 65.7% of breast cancers were
classified as high-score tumours, whereas 8.5% were
classified as low-score tumours with abnormality
scores <30; these low-score tumours were regarded
as false-negative cases. Table 1 presents the medians
and interquartile ranges of abnormality scores
according to clinical, radiological, and pathological
phenotypes.
Palpable lesions, cancers in entirely fatty or
scattered fibroglandular breasts, cancers presenting
as masses with or without calcifications and
architectural distortion, and larger cancers were
associated with higher abnormality scores (all
P<0.001) [Table 1]. Invasive cancers had higher abnormality scores compared with ductal carcinoma
in situ (P=0.010). Axillary nodal status (P=0.078)
and cancer molecular subtype (P=0.820) were not
associated with abnormality scores (Table 2).
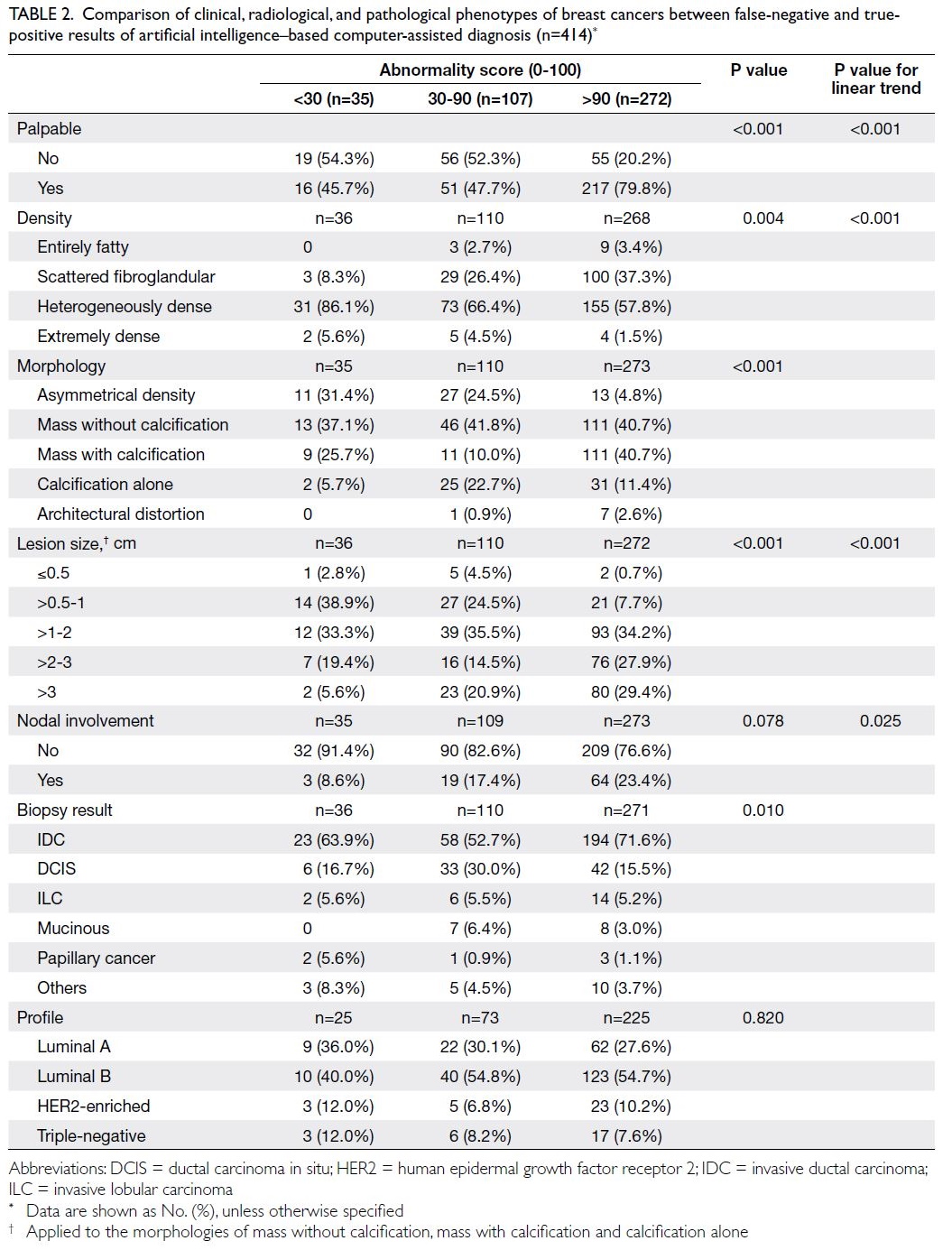
Table 2. Comparison of clinical, radiological, and pathological phenotypes of breast cancers between false-negative and truepositive results of artificial intelligence–based computer-assisted diagnosis (n=414)
Phenotypic features of high-score breast
cancer
High-score breast cancers had higher prevalences of
palpable disease, cancers presenting as masses with
or without calcifications, invasive cancers, and larger
cancers (>1 cm) [Table 2].
Phenotypic features of low-score, false-negative
breast cancer
The false-negative rate for AI-CAD was 8.5%
(35/414). These cancers had higher prevalences
of non-palpable disease, cancers presenting as
asymmetrical densities, small cancers (<1 cm), and
locations in heterogeneously dense or extremely
dense breast tissue.
Impact of tumour-to–breast area ratio
and tumour distance from chest wall on abnormality score
Overall, 158 cancers presenting as masses without
calcifications were included in this analysis. The
mean abnormality score for cancers with a TBAR
of ≥30% was significantly lower than for those with
a TBAR of <30% (86.7 vs 54.4; P<0.001). Tumours
bordering the chest wall (ie, distance of 0 cm
from chest wall) demonstrated significantly lower abnormality scores compared with those located 1 cm and ≥2 cm away from the chest wall (mean, 65.5 vs 89.2 vs 87.2; P<0.001).
Distribution of abnormality scores for
unaffected breasts
In the analysis of 347 unaffected breasts (regarded
as negative findings by reporting radiologists), the
median abnormality score was 0 (mean, 3.5; range,
0-81). Using a threshold score of 30, the false-positive
rate was 3.7% (13/347), indicating 96.3% specificity.
Most of these false positives (11/13) scored between
30 and 50; none scored >90. One case with known
postoperative changes from breast conservative
surgery showed stable mammographic finding for
10 years, scored 81 by AI-CAD. One case with a
breast cyst scored 73, which was confirmed via fine
needle aspiration cytology.
Discussion
Performance and potentials
Most AI-CAD algorithms provide heatmaps with
abnormality scores ranging from 0 to 100; a higher
score generally implies a greater likelihood of
cancer. Previous AI-CAD studies have used various
threshold scores; some set a threshold of 10 for
population screening,18 19 20 whereas Weigel et al21 set a
threshold of 28 for detecting malignant calcifications.
However, the clinical implications of the abnormality
score itself have not been clarified; a score range
from 10 to 100 may be too broad for distinguishing
malignancies in clinical practice. These aspects
highlight the need for further validation of the
appropriate reference score provided by AI-CAD
algorithms. In this study, we set the threshold at 30
because, unlike population screening approaches,
our patients were symptomatic individuals. A higher
threshold score appears more practical in the clinical
setting of symptomatic patients.
In our study, the AI-CAD algorithm detected
91.5% (379/414) of breast cancers with an abnormality
score of >30; of these 379 cancers, 71.7% exhibited
a high abnormality score of >90. The false-negative
rate of 8.5% is comparable to previously reported
rate for this AI-CAD algorithm.5
All cancers presenting as architectural
distortion in our study were correctly localised by
the AI-CAD, with abnormality scores >30; 87.5% of
them were assigned high abnormality scores of >90
(Fig 4a and b). Unlike cancers presenting as masses
or calcifications, cancers presenting as architectural
distortion remain challenging for radiologists to
detect and interpret.22 23 24 Wan et al25 showed that a standalone AI algorithm did not outperform
radiologists; however, with AI assistance, junior
radiologists demonstrated significant improvements
in diagnostic accuracy for architectural distortion.
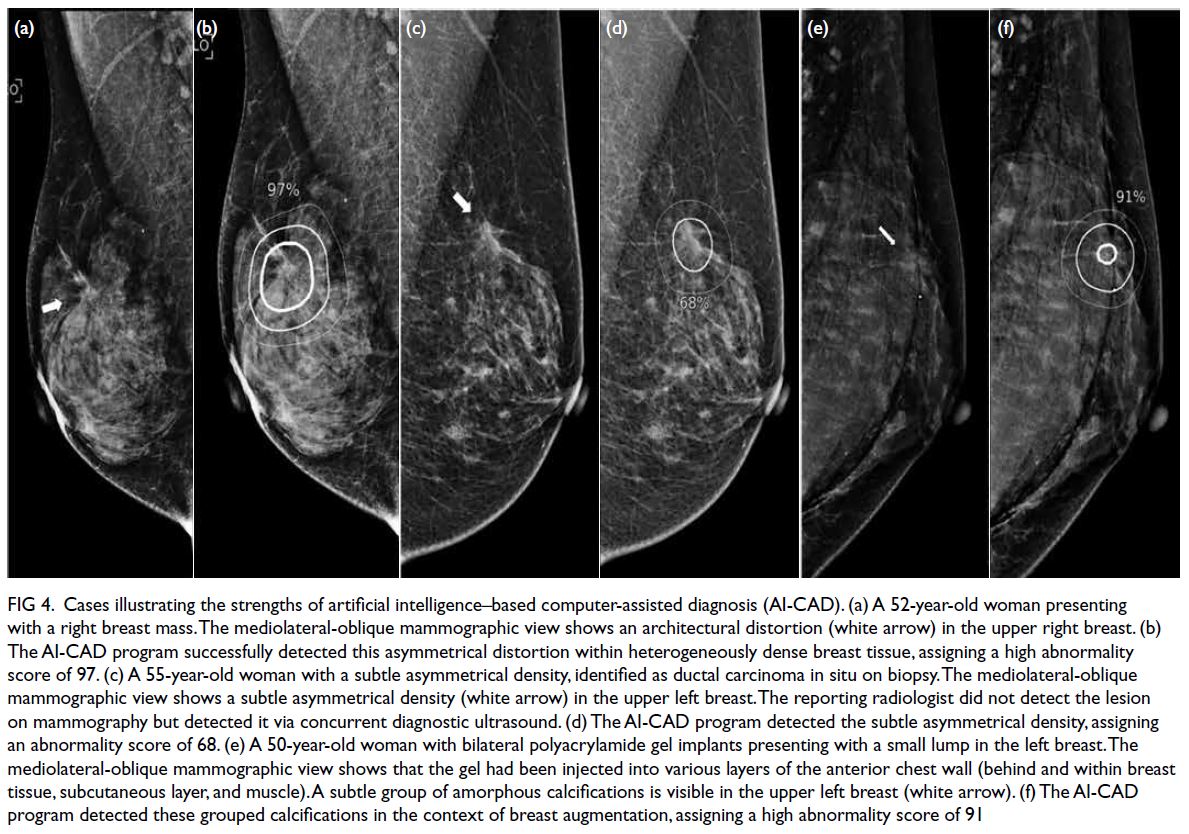
Figure 4. Cases illustrating the strengths of artificial intelligence–based computer-assisted diagnosis (AI-CAD). (a) A 52-year-old woman presenting with a right breast mass. The mediolateral-oblique mammographic view shows an architectural distortion (white arrow) in the upper right breast. (b) The AI-CAD program successfully detected this asymmetrical distortion within heterogeneously dense breast tissue, assigning a high abnormality score of 97. (c) A 55-year-old woman with a subtle asymmetrical density, identified as ductal carcinoma in situ on biopsy. The mediolateral-oblique mammographic view shows a subtle asymmetrical density (white arrow) in the upper left breast. The reporting radiologist did not detect the lesion on mammography but detected it via concurrent diagnostic ultrasound. (d) The AI-CAD program detected the subtle asymmetrical density, assigning an abnormality score of 68. (e) A 50-year-old woman with bilateral polyacrylamide gel implants presenting with a small lump in the left breast. The mediolateral-oblique mammographic view shows that the gel had been injected into various layers of the anterior chest wall (behind and within breast tissue, subcutaneous layer, and muscle). A subtle group of amorphous calcifications is visible in the upper left breast (white arrow). (f) The AI-CAD program detected these grouped calcifications in the context of breast augmentation, assigning a high abnormality score of 91
One case of breast cancer presenting as
asymmetric density in heterogeneously dense breast
tissue was missed by the reporting radiologist but
detected by AI-CAD, which assigned an abnormality
score of 68. The cancer was later identified by the radiologist via ultrasound, which is part of routine
workup for symptomatic patients in our centre.
Retrospective review indicated that the asymmetric
density was visible on mammography (Fig 4c and d).
In a study by Kim et al,26 40 of 128 mammographically occult breast cancers were correctly identified by
the AI algorithm, demonstrating its added value in
detecting such cancers.
The 64 cases of mammographically
occult breast cancer not detected by either the
AI-CAD or the radiologists were excluded from
the study. Of these cases, 84.3% were found in
heterogeneously dense and extremely dense breast
tissue (BI-RADS 3 and 4).16 Dense breast tissue is
recognised as a significant feature associated with
mammographically occult and missed cancers.27 28 29 30
We suspect that mammographic signs of cancer are
masked or obscured by dense breast parenchyma,
thus evading detection by the AI-CAD. Conversely,
both radiologists and the AI-CAD tended to more
effectively detect cancers in fatty breasts.18
In our study, the AI-CAD correctly localised
a small breast cancer with a high abnormality score
(>90) in a patient with polyacrylamide hydrogel
(PAAG)–injected augmentation mammoplasty
(Fig 4e and f). The diagnosis of breast cancer after
PAAG-injected augmentation mammoplasty is
challenging. Lesion visualisation may be masked by the presence of polyacrylamide gel, and
extravasated polyacrylamide gel may mimic a lesion
on mammography, potentially delaying early cancer
detection. In such cases, assessments of suspicious
calcifications and parenchymal distortion within
visible breast parenchyma are considered the
main goals of screening mammography.31 32 The
effectiveness of AI-CAD in detecting breast cancer
among patients with augmentation mammaplasty
remains uncertain, warranting further studies.
Detection challenges and future directions
Isolated cases of large, clearly visible lesions that
evaded AI detection have been described by Lång
et al33 and Choi et al.18 To our knowledge, our study
is the first to investigate factors contributing to
such evasion. In this study, the AI algorithm tended
to underscore cancers presenting as large masses
(Fig 5a and b). Cancers with a TBAR of ≥30% had
significantly lower mean abnormality scores relative
to those with a ratio of <30%. Tumours bordering the
chest wall (0 cm distance) also showed significantly
lower abnormality scores than those located away from the chest wall. The underlying cause remains
unclear; however, these findings highlight concerns
regarding the use of AI-CAD as a standalone tool
for triaging cases in symptomatic populations. We
also noted that the AI-CAD missed certain cancers
with obvious findings, such as nipple retraction and
diffuse dermal thickening (Fig 5c to f).
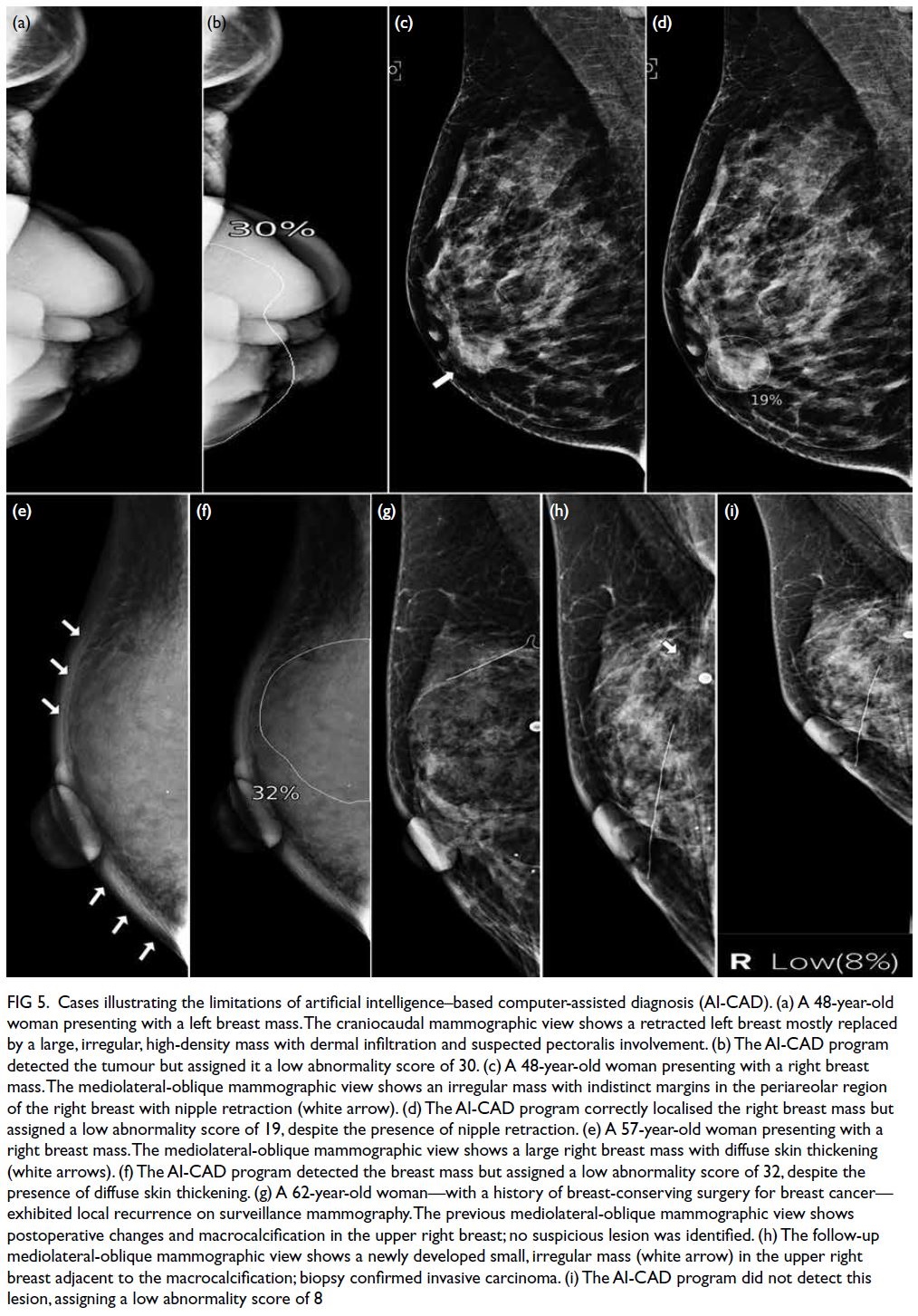
Figure 5. Cases illustrating the limitations of artificial intelligence–based computer-assisted diagnosis (AI-CAD). (a) A 48-year-old woman presenting with a left breast mass. The craniocaudal mammographic view shows a retracted left breast mostly replaced by a large, irregular, high-density mass with dermal infiltration and suspected pectoralis involvement. (b) The AI-CAD program detected the tumour but assigned it a low abnormality score of 30. (c) A 48-year-old woman presenting with a right breast mass. The mediolateral-oblique mammographic view shows an irregular mass with indistinct margins in the periareolar region of the right breast with nipple retraction (white arrow). (d) The AI-CAD program correctly localised the right breast mass but assigned a low abnormality score of 19, despite the presence of nipple retraction. (e) A 57-year-old woman presenting with a right breast mass. The mediolateral-oblique mammographic view shows a large right breast mass with diffuse skin thickening (white arrows). (f) The AI-CAD program detected the breast mass but assigned a low abnormality score of 32, despite the presence of diffuse skin thickening. (g) A 62-year-old woman—with a history of breast-conserving surgery for breast cancer— exhibited local recurrence on surveillance mammography. The previous mediolateral-oblique mammographic view shows postoperative changes and macrocalcification in the upper right breast; no suspicious lesion was identified. (h) The follow-up mediolateral-oblique mammographic view shows a newly developed small, irregular mass (white arrow) in the upper right breast adjacent to the macrocalcification; biopsy confirmed invasive carcinoma. (i) The AI-CAD program did not detect this lesion, assigning a low abnormality score of 8
Moreover, the inability of AI-CAD to compare
mammograms with previous studies may hinder
its effectiveness in specific scenarios, such as the
detection of subtle developing symmetries and
identification of early recurrence in postoperative
cases (Fig 5g to i). In contrast, radiologists can
compare mammograms with previous studies,
improving mammogram interpretation accuracy.
Studies have shown that the diagnostic
performances of AI algorithms are comparable to
those of radiologists in terms of assessing screening
mammograms; the use of AI to triage screening
mammograms could potentially reduce radiologists’
workload.5 34 35 We identified potential limitations
and weaknesses of AI-CAD in diagnosing breast
cancers under certain conditions, highlighting the
need for further large-scale studies to investigate
clinical applications of AI-CAD in symptomatic
patients.
Strengths and limitations
This study had several key strengths. To our
knowledge, it is the first to evaluate AI-CAD for
breast cancer detection in Hong Kong, using an
AI-CAD system that had not previously been
exposed to images from our centres during their
product development. Additionally, all digital
mammograms were obtained before biopsies,
avoiding any biopsy-related changes which could
potentially affect AI-CAD performance. Limitations
of the study include its retrospective design and
inclusion of cancer-enriched datasets, which may
lead to overestimation of AI-CAD performance;
the use of a single AI vendor, hindering applicability
to other AI algorithms; and the lack of BI-RADS
correlation. Furthermore, there was a lack of
information concerning progression in unaffected
breasts over an extended follow-up interval (≥2
years), which could impact the false-positive rate
of the AI-CAD. An extended observation period is
needed to identify potential malignancies that may
have been initially missed by radiologists.
Conclusion
Unlike other developed cities or countries, Hong
Kong does not have population-based screening
programmes. The adoption and implementation
of AI programs in Hong Kong for breast imaging
remains in early stages, mainly due to ongoing
debates about efficacy and a lack of sufficient local data to support widespread application. Current
literature is almost entirely based on population
screening data, which may not be applicable to
cities without screening programmes. In our
study, AI-CAD demonstrated promising accuracy
in detecting breast cancers within symptomatic
settings; its performance varied according to radio-pathological
characteristics. To translate these
research findings into practical clinical applications,
further validation studies with larger sample sizes
are required; these would confirm the reliability of
AI-CAD systems. The development of protocols for
integrating AI-CAD into existing clinical workflows,
formulation of usage guidelines, and initiation of
training programmes for radiologists to effectively
utilise AI as a second reader are essential elements
of this process. Collaborations with information
technology departments and hospital management
are necessary to ensure successful integration.
Although further investigation is needed, this
study provides encouraging evidence to support
the use of AI-CAD as a breast cancer detection
tool in symptomatic settings, ultimately benefitting
patients.
Author contributions
Concept or design: SM Yu, MNY Choi, EHY Hung, HHL Chau.
Acquisition of data: SM Yu, MNY Choi, TH Chan, CYM Young, YH Chan, YS Chan, C Tsoi.
Analysis or interpretation of data: SM Yu, TH Chan, J Leung.
Drafting of the manuscript: SM Yu, CYM Young.
Critical revision of the manuscript for important intellectual content: SM Yu, CYM Young, WCW Chu, EHY Hung, HHL Chau.
Acquisition of data: SM Yu, MNY Choi, TH Chan, CYM Young, YH Chan, YS Chan, C Tsoi.
Analysis or interpretation of data: SM Yu, TH Chan, J Leung.
Drafting of the manuscript: SM Yu, CYM Young.
Critical revision of the manuscript for important intellectual content: SM Yu, CYM Young, WCW Chu, EHY Hung, HHL Chau.
All authors had full access to the data, contributed to the study, approved the final version for publication, and take responsibility for its accuracy and integrity.
Conflicts of interest
All authors have disclosed no conflicts of interest.
Funding/support
This research received no specific grant from any funding agency in the public, commercial, or not-for-profit sectors.
Ethics approval
This research was approved by the New Territories East Cluster Research Ethics Committee/Institutional Review
Board of Hospital Authority, Hong Kong (Ref No.: NTEC-2023-074). The requirement for informed patient consent was waived by the Committee due to the retrospective nature of the research.
References
1. Tabár L, Vitak B, Chen HH, Yen MF, Duffy SW, Smith RA.
Beyond randomized controlled trials: organized
mammographic screening substantially reduces breast
carcinoma mortality. Cancer 2001;91:1724-31. Crossref
2. Majid AS, de Paredes ES, Doherty RD, Sharma NR,
Salvador X. Missed breast carcinoma: pitfalls and pearls.
Radiographics 2003;23:881-95. Crossref
3. Fenton JJ, Taplin SH, Carney PA, et al. Influence of
computer-aided detection on performance of screening
mammography. N Engl J Med 2007;356:1399-409. Crossref
4. Lehman CD, Wellman RD, Buist DS, et al. Diagnostic
accuracy of digital screening mammography with and
without computer-aided detection. JAMA Intern Med
2015;175:1828-37. Crossref
5. Dembrower K, Wåhlin E, Liu Y, et al. Effect of artificial
intelligence–based triaging of breast cancer screening
mammograms on cancer detection and radiologist
workload: a retrospective simulation study. Lancet Digit
Health 2020;2:e468-74. Crossref
6. Raya-Povedano JL, Romero-Martín S, Elías-Cabot E,
Gubern-Mérida A, Rodríguez-Ruiz A, Álvarez-Benito M.
AI-based strategies to reduce workload in breast cancer
screening with mammography and tomosynthesis: a
retrospective evaluation. Radiology 2021;300:57-65. Crossref
7. Erickson BJ, Korfiatis P, Kline TL, Akkus Z, Philbrick K,
Weston AD. Deep learning in radiology: does one size fit
all? J Am Coll Radiol 2018;15(3 Pt B):521-6. Crossref
8. Schünemann HJ, Lerda D, Quinn C, et al. Breast cancer
screening and diagnosis: a synopsis of the European breast
guidelines. Ann Intern Med 2020;172:46-56. Crossref
9. Bahl M. Artificial intelligence: a primer for breast imaging
radiologists. J Breast Imaging 2020;2:304-14. Crossref
10. Hickman SE, Woitek R, Le EP, et al. Machine learning
for workflow applications in screening mammography:
systematic review and meta-analysis. Radiology
2022;302:88-104. Crossref
11. Leibig C, Brehmer M, Bunk S, Byng D, Pinker K, Umutlu L.
Combining the strengths of radiologists and AI for breast
cancer screening: a retrospective analysis. Lancet Digit
Health 2022;4:e507-19. Crossref
12. Intelligence Unit, The Economist. Breast cancer in Asia—the challenge and response. A report from the Economist Intelligence Unit. 2016. Available from: https://www.eiuperspectives.economist.com/sites/default/files/EIU Breast Cancer in Asia_Final.pdf. Accessed 19 Nov 2017.
13. Lim YX, Lim ZL, Ho PJ, Li J. Breast cancer in Asia:
incidence, mortality, early detection, mammography
programs, and risk-based screening initiatives. Cancers
(Basel) 2022;14:4218. Crossref
14. Salim M, Wåhlin E, Dembrower K, et al. External evaluation
of 3 commercial artificial intelligence algorithms for
independent assessment of screening mammograms.
JAMA Oncol 2020;6:1581-8. Crossref
15. Kim HE, Kim HH, Han BK, et al. Changes in cancer
detection and false-positive recall in mammography using
artificial intelligence: a retrospective, multireader study.
Lancet Digit Health 2020;2:e138-48. Crossref
16. D’Orsi CJ, Sickles EA, Mendelson EB, et al. ACR BIRADS®
Atlas, Breast Imaging Reporting and Data System. Reston,
VA: American College of Radiology; 2013.
17. McKinney SM, Sieniek M, Godbole V, et al. International
evaluation of an AI system for breast cancer screening.
Nature 2020;577:89-94. Crossref
18. Choi WJ, An JK, Woo JJ, Kwak HY. Comparison of
diagnostic performance in mammography assessment:
radiologist with reference to clinical information versus
standalone artificial intelligence detection. Diagnostics (Basel) 2022;13:117. Crossref
19. Lee SE, Han K, Yoon JH, Youk JH, Kim EK. Depiction
of breast cancers on digital mammograms by artificial
intelligence–based computer-assisted diagnosis according
to cancer characteristics. Eur Radiol 2022;32:7400-8. Crossref
20. Koch HW, Larsen M, Bartsch H, Kurz KD, Hofvind S.
Artificial intelligence in BreastScreen Norway: a
retrospective analysis of a cancer-enriched sample including
1254 breast cancer cases. Eur Radiol 2023;33:3735-43. Crossref
21. Weigel S, Brehl AK, Heindel W, Kerschke L. Artificial
intelligence for indication of invasive assessment of
calcifications in mammography screening [in English,
German]. Rofo 2023;195:38-46. Crossref
22. Suleiman WI, McEntee MF, Lewis SJ, et al. In the digital era,
architectural distortion remains a challenging radiological
task. Clin Radiol 2016;71:e35-40. Crossref
23. Babkina TM, Gurando AV, Kozarenko TM, Gurando VR,
Telniy VV, Pominchuk DV. Detection of breast cancers
represented as architectural distortion: a comparison
of full-field digital mammography and digital breast
tomosynthesis. Wiad Lek 2021;74:1674-9. Crossref
24. Alshafeiy TI, Nguyen JV, Rochman CM, Nicholson BT,
Patrie JT, Harvey JA. Outcome of architectural distortion
detected only at breast tomosynthesis versus 2D
mammography. Radiology 2018;288:38-46. Crossref
25. Wan Y, Tong Y, Liu Y, et al. Evaluation of the combination
of artificial intelligence and radiologist assessments
to interpret malignant architectural distortion on
mammography. Front Oncol 2022;12:880150. Crossref
26. Kim HJ, Kim HH, Kim KH, et al. Mammographically
occult breast cancers detected with AI-based diagnosis
supporting software: clinical and histopathologic
characteristics. Insights Imaging. 2022;13:57. Crossref
27. Lian J, Li K. A review of breast density implications and
breast cancer screening. Clin Breast Cancer 2020;20:283-90. Crossref
28. Freer PE. Mammographic breast density: impact on breast
cancer risk and implications for screening. Radiographics
2015;35:302-15. Crossref
29. Arora N, King TA, Jacks LM, et al. Impact of breast density
on the presenting features of malignancy. Ann Surg Oncol
2010;17 Suppl 3:211-8. Crossref
30. Ma L, Fishell E, Wright B, Hanna W, Allan S, Boyd NF.
Case-control study of factors associated with failure to
detect breast cancer by mammography. J Natl Cancer Inst
1992;84:781-5. Crossref
31. Cheng NX, Liu LG, Hui L, Chen YL, Xu SL. Breast
cancer following augmentation mammaplasty with
polyacrylamide hydrogel (PAAG) injection. Aesthetic Plast
Surg 2009;33:563-69. Crossref
32. Teo SY, Wang SC. Radiologic features of polyacrylamide
gel mammoplasty. AJR Am J Roentgenol 2008;191:W89-95. Crossref
33. Lång K, Dustler M, Dahlblom V, Åkesson A, Andersson I,
Zackrisson S. Identifying normal mammograms in a large
screening population using artificial intelligence. Eur
Radiol 2021;31:1687-92. Crossref
34. Rodriguez-Ruiz A, Lång K, Gubern-Merida A, et al. Stand-alone
artificial intelligence for breast cancer detection in
mammography: comparison with 101 radiologists. J Natl
Cancer Inst 2019;111:916-22. Crossref
35. Rodríguez-Ruiz A, Krupinski E, Mordang JJ, et al. Detection
of breast cancer with mammography: effect of an artificial
intelligence support system. Radiology 2019;290:305-14. Crossref