Hong Kong Med J 2018 Apr;24(2):137–44 | Epub 6 Apr 2018
DOI: 10.12809/hkmj177118
© Hong Kong Academy of Medicine. CC BY-NC-ND 4.0
ORIGINAL ARTICLE
Reduction of operative mortality after implementation
of Surgical Outcomes Monitoring and Improvement Programme by Hong Kong
Hospital Authority
WC Yuen, FHKAM (Surgery)1; K Wong, MSc2;
YS Cheung, FHKAM (Surgery)3; Paul BS Lai, FHKAM (Surgery)3
1 Department of Surgery, Ruttonjee and
Tang Shiu Kin Hospital, Hospital Authority, Hong Kong
2 Jockey Club School of Public Health
and Primary Care, Faculty of Medicine, The Chinese University of Hong
Kong, Shatin, Hong Kong
3 Department of Surgery, Prince of Wales
Hospital, The Chinese University of Hong Kong, Shatin, Hong Kong
Corresponding author: Prof Paul BS Lai (paullai@surgery.cuhk.edu.hk)

Abstract
Introduction: Since 2008, the
Hong Kong Hospital Authority has implemented a Surgical Outcomes
Monitoring and Improvement Programme (SOMIP) at 17 public hospitals with
surgical departments. This study aimed to assess the change in operative
mortality rate after implementation of SOMIP.
Methods: The SOMIP included all Hospital Authority patients undergoing major/ultra-major procedures in general surgery, urology, plastic surgery, and paediatric surgery. Patients undergoing liver or renal transplantation or who had multiple trauma or massive bowel ischaemia were excluded. In SOMIP, data retrieval from the Hospital Authority patient database was performed by six full-time nurse reviewers following a set of precise data definitions. A total of 230 variables were collected for each patient, on demographics, preoperative and operative variables, laboratory test results, and postoperative complications up to 30 days after surgery. In this study, we used SOMIP cumulative 5-year data to generate risk-adjusted 30-day mortality models by hierarchical logistic regression for both emergency and elective operations. The models expressed overall performance as an annual observed-to-expected mortality ratio.
Results: From 2009/2010 to
2015/2016, the overall crude mortality rate decreased from 10.8% to 5.6%
for emergency procedures and from 0.9% to 0.4% for elective procedures.
From 2011/2012 to 2015/2016, the risk-adjusted observed-to-expected
mortality ratios showed a significant downward trend for both emergency
and elective operations: from 1.126 to 0.796 and from 1.150 to 0.859,
respectively (Mann-Kendall statistic = –0.8; P<0.05 for both).
Conclusion: The Hospital
Authority’s overall crude mortality rates and risk-adjusted
observed-to-expected mortality ratios for emergency and elective
operations significantly declined after SOMIP was implemented.
New knowledge added by this study
- A Surgical Outcomes Monitoring and Improvement Programme allows monitoring of performance and fair comparison of individual Hospital Authority hospitals against the overall Hospital Authority average. It enhances the understanding of surgical performance and helps identify areas for improvement.
- The Hospital Authority’s overall crude mortality rates and risk-adjusted observed-to-expected mortality ratios for emergency and elective operations significantly declined after SOMIP was implemented.
- A properly organised, risk-adjusted clinical audit can accurately measure surgical outcomes and provide information for surgeons to deliver quality improvement.
Introduction
Audits of surgical mortality are used worldwide to
monitor surgical outcome and achieve quality assurance.1 By measuring and comparing properly collected,
risk-adjusted surgical outcome data, quality of surgical care could be
enhanced in participating institutions.2
It has been demonstrated in several countries that adoption of a national
surgical audit programme can reduce mortality.2
3
The Hong Kong Hospital Authority (HA) was
established in 1991. It is a government statutory body responsible for the
management of 42 public hospitals and institutions, 47 specialist
out-patient clinics, and 73 general out-patient clinics in Hong Kong.
Seventeen HA hospitals have surgical departments; all of them provide an
elective surgery service and 14 also provide an emergency surgical
service. In 2016, over 80% of all hospital admissions in Hong Kong were
under the management of the HA.4
Therefore, it is important for the HA to develop tools with which to
measure and improve performance. For this purpose, the HA Coordinating
Committee of Surgery set up a Central Surgical Audit Unit in 2001. From
2002 to 2007, the unit conducted clinical audits based on retrospective
cumulative data to compare the performance of the 17 surgical departments.
One to two major or ultra-major operations, such as major hepatectomy,
oesophagectomy, and major lung resection, were selected each year for
comparison. The risk-adjustment model used was based on the Physiological
and Operative Severity Score for Enumeration of Mortality and Morbidity
(POSSUM).5 6 Those audits were procedure-based and mainly focused on
mortality, and hence a more comprehensive risk-adjusted outcome audit was
needed.
In 2008, a new programme—namely, the Surgical
Outcomes Monitoring and Improvement Programme (SOMIP)—was launched by the
HA with the aim of monitoring and improving surgical quality. The
programme was governed by a steering committee comprising surgeons, an
anaesthetist, a physician, administrative managers, and statisticians.
Risk-adjusted mortality and morbidity rates of elective and emergency
major and ultra-major operations at each hospital are measured and
reported yearly. An annual forum is held to disseminate the results and
allow the sharing of best practices.
This study aimed to assess the changes in overall
surgical outcomes for patients after implementation of SOMIP.
Methods
Between July 2008 and June 2016, SOMIP captured
data of all elective and emergency major/ultra-major operations (except
those in children younger than 1 year) that were performed by general
surgery, urology, plastic and reconstructive surgery, and paediatric
surgery teams at all 17 HA hospitals. A total of 230 variables were
collected from each patient: 10 patient demographic variables, 83
preoperative and operative variables, 31 laboratory test results, and 40
postoperative events and 66 postoperative adverse events in the first 30
days after surgery. Demographic data and laboratory test results were
mostly automatically retrieved from various HA clinical information
systems. For data that required manual retrieval, six full-time SOMIP
nurse reviewers were employed by the HA head office for this purpose.
Preoperative and operative variables, as well as postoperative
complications occurring up to 30 days after the index operation, were
retrieved from patient records by the SOMIP nurse reviewers. Mortality at
30 days, 60 days, and 90 days were also retrieved from the HA electronic
database. These data were endorsed and submitted by each surgical
department’s surgical supervisor within 60 days of surgery.
Both the manually captured and automatically
captured data were entered into a tailor-made SOMIP electronic database.
Data variable definitions were listed in the operation manual of the
programme. To ensure data validity and consistency, all nurse reviewers
completed comprehensive training on data definition and criteria, and
regular nurse reviewer meetings were held to clarify any queries. All data
were endorsed by the surgical supervisor of the respective surgical
department. When necessary, data definitions were modified.
An inter-rater reliability test was performed each
May and completed within a month so as to ensure consistency among nurse
reviewers as well as data accuracy. Fifty cases were sampled for
evaluation using a stratified systematic sampling method. For those
selected cases, an independent nurse from the SOMIP team repeated the data
collection using a designated data template, without prior knowledge of
the information recorded by the original nurse reviewer. Data quality was
measured by comparing two sets of data, and inferred by a score defined as
the percentage of agreement between nurse reviewer and the SOMIP working
team for each data item. The mean score of all data items was used to
assess overall performance. The overall result was satisfactory and the
mean score of all data items was 99.3% (range of individual item scores,
95.2%-100%).
Among the variables collected, preoperative risk
factors including demographic data; general health and lifestyle
variables; and major respiratory, cardiovascular, hepatobiliary, renal,
vascular, central nervous system, and immune co-morbidities were deemed
particularly important. These risk factors were modified from those in the
American College of Surgeons National Surgical Quality and Improvement
Program (NSQIP) to suit the local context.7
8 9
Operative variables included intra-operative blood loss, American Society
of Anesthesiologists physical status classification, procedure complexity score, surgical
subspecialty, wound classification, operative magnitude, and operative
time. Before analysis and reporting, SOMIP data were cleaned and verified
by four surgeons and the SOMIP surgical supervisors of each hospital.
Questionable cases were reviewed accordingly. Cases of liver
transplantation, renal transplantation, multiple trauma, and major bowel
ischaemia (Table 1)10 11 12
13 14
15 16
17 were excluded from the
risk-adjusted model analysis.
Comparative risk-adjusted models (different models
for emergency operations and elective operations) were generated using
hierarchical logistic regression. The 30-day risk-adjusted mortality
models expressed hospital performance as expected odds ratios. A
risk-adjusted observed-to-expected mortality ratio (O/E ratio) was then
calculated for each hospital. The O/E ratio is a quotient between the
observed number of deaths and the expected number of deaths; the latter
was calculated by a logistic regression method based on significant
independent risk factors. Together with the 90% confidence intervals, O/E
ratios were depicted by caterpillar plots and boxplots. Hospitals with the
lower limit of the 90% confidence interval of O/E ratios greater than 1
were defined as ‘high outliers’; hospitals with the upper limit of the 90%
confidence interval of O/E ratios lower than 1 were defined as ‘low
outliers’. The risk-adjusted outcome of a ‘high-outlier’ hospital was
probably worse than the average outcome, and that of a ‘low-outlier’
hospital was probably better than the average outcome.
In addition to risk-adjusted postoperative
mortality, various general medical and surgical complications, as well as
specific complications (anastomotic leakage, surgical site infection,
acute myocardial infarction, pneumonia), were recorded. The list of
complications recorded and the method to derive the SOMIP risk-adjustment
model have been described in detail in the annual SOMIP Report.10 11 12 13 14 15 16 17 Different
levels of confidence were used for different outcome variables—90%
confidence interval for mortality rates, 95% confidence interval for major
complications, and 99% confidence interval for morbidity rates. Using
SOMIP data together with other useful information extracted from the HA
Executive Information System (eg, bed occupancy, nursing manpower,
intensive care unit support, and surgeon workload), root-cause analyses
were performed using multilevel logistic regression, as described in the
annual SOMIP Report.10 11 12 13 14 15 16 17
The discriminative power of the risk-adjusted
models was measured by the C-index, area under the receiver-operating
characteristic curve (AUC). The closer the C-index is to 1, the better the
discriminative power of the model is: a C-index of ≥0.8 indicates
excellent discriminative power.18
The calibration accuracy of the models was assessed by the Hosmer-Lemeshow
goodness-of-fit test (HL test). The calibration of the model was rejected
if P<0.05. The Mann-Kendall non-parametric trend test was used to
identify trends (positive or negative) in the annual data series for both
crude mortality rates (2009/2010 to 2015/2016) and risk-adjusted O/E
ratios (2011/2012 to 2015/2016). A very high positive value of the
Mann-Kendall statistic (S) indicated an increasing trend; a very
low negative value indicated a decreasing trend. The test statistic Z-score
was used as a measure of trend significance.
Results
Descriptive data
Age distribution trends are summarised in Table
2.10 11 12 13 14 15 16 17 From 2008/2009 to 2015/2016, the proportion of people
aged 61-70 years increased by 7 percentage points (from 19% to 26%),
whereas the proportions of people aged 41-50 years and 71-80 years
decreased by 2 and 5 percentage points, respectively.
Table 310 11 12
13 14
15 16
17 shows proportions of patients
taking regular medication for diabetes mellitus and hypertension before
surgery from 2009/2010 to 2015/2016, as well as those currently smoking
(within 1 year) and drinking more than 2 units of alcohol per day in the
previous 2 weeks. Over 70% of patients had at least one of these four
conditions, whereas about 40% had a history of regular use of hypertension
medication before surgery.
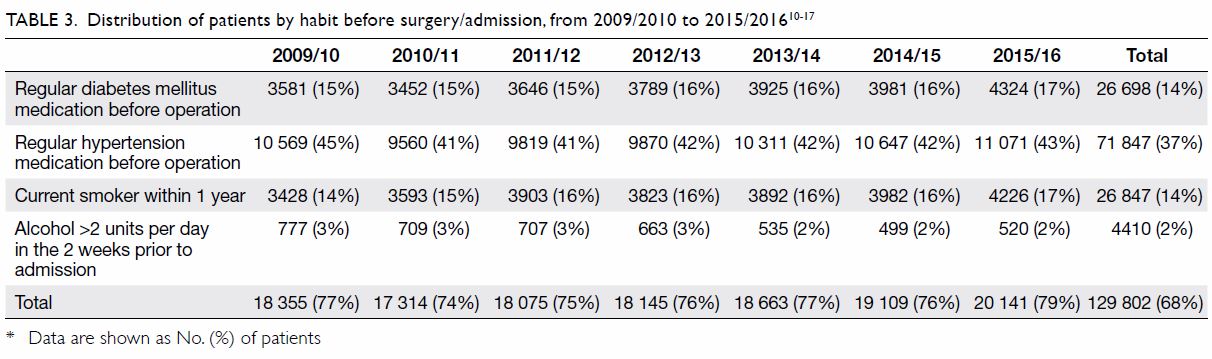
Table 3. Distribution of patients by habit before surgery/admission, from 2009/2010 to 2015/201610 11 12 13 14 15 16 17
Annual numbers of elective operations by specialty
are summarised in Table 4.10 11 12
13 14
15 16
17 For the 10 listed elective
operations, the most frequently performed were in urology, consistently
constituting 28% of the caseload from 2008/09 to 2015/16. The least
frequently performed procedures were parotid surgery (1%) and paediatric
surgery (1%).
Overall crude mortality rates and risk-adjusted
observed-to-expected mortality ratios
From July 2008 to June 2016, eight SOMIP reports
were published.10 11 12 13 14 15 16 17 They showed that the HA overall crude mortality rate
approximately halved over this time. The crude 30-day mortality rate for
emergency operations dropped gradually from 10.8% in the year 2009/2010 to
5.6% in 2015/2016 (Fig 1). Similarly, the crude 30-day mortality rate
for elective operations more than halved: from 0.9% in 2009/2010 to 0.4%
in 2015/2016 (Fig 2).
In the 5-year cumulative comparison analysis
(2011/2012 to 2015/2016), both models had excellent discriminative power
and good calibration accuracy. For emergency operations, the AUC was
>0.9 and the HL test statistic was >0.1; for elective operations,
the AUC was >0.89 and the HL test statistic was >0.2. The
risk-adjusted observed-to-expected 30-day mortality ratio for both types
of surgery showed a similar downward trend to the crude mortality rates.
For emergency operations, the risk-adjusted O/E ratios were 1.126, 1.022,
1.113, 0.921, and 0.796 across the 5 years (Fig 3). These values show a statistically
significant downward (negative) trend (S= –0.8; P<0.05). The results of
the Mann-Kendall analyses are summarised in Table 4. For elective operations, risk-adjusted O/E
ratios were 1.150, 1.229, 0.881, 0.862, and 0.859 across the 5 years (Fig
4). These values show a statistically significant downward
(negative) trend (S= –0.8; P<0.05) [Table 5].
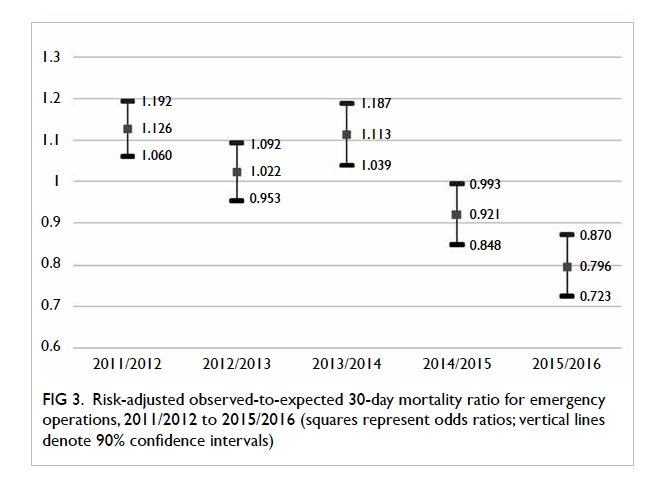
Figure 3. Risk-adjusted observed-to-expected 30-day mortality ratio for emergency operations, 2011/2012 to 2015/2016
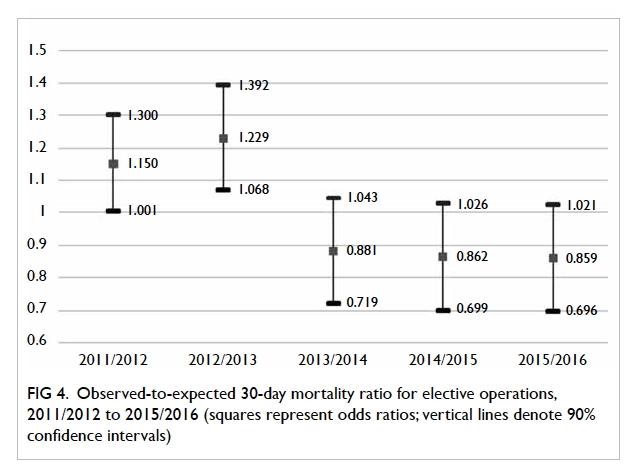
Figure 4. Observed-to-expected 30-day mortality ratio for elective operations, 2011/2012 to 2015/2016
Discussion
Before the turn of the century, most hospital
records in HA hospitals were handwritten and retained by individual
hospitals. There was no convenient means by which to share patient details
among hospitals. Around 2001, the HA implemented a number of clinical
management electronic systems, such as the electronic patient record,
Operation Theatre Record System, and Clinical Data Analysis and Reporting
System, at all HA hospitals. By virtue of this infrastructure, patient
records and information about diagnoses and operations could be accessed
at a central level. Based on this central clinical database, a Quality
Assurance Subcommittee under the Coordinating Committee of Surgery
commenced small-scale comparative clinical audits for ultra-major
operations in 2002, focusing on one to two ultra-major operations per
year. The audits provided basic information about hospital performance for
the selected operation, such as number of procedures, age distribution of
patients, and mortality rate.
Subsequently, the Coordinating Committee of Surgery
developed a more robust system to monitor more major operations at the
same time. It was decided to follow the framework of NSQIP, which was
developed in 1994 by the Veterans Affairs Hospitals in the United States
to monitor risk-adjusted surgical operation outcomes. Like HA hospitals,
Veterans Affairs Hospitals are managed by a central governing body and
equipped with a comprehensive electronic medical records system. Studies19 20
showed a significant improvement in both mortality and morbidity over time
and thus, in 2004, NSQIP was extended to private hospitals and endorsed by
the American College of Surgeons. Using NSQIP as the blueprint, the HA
launched SOMIP in 2008. The SOMIP adopted similar risk-adjustment
variables, use of nurse reviewers to collect data, a focus on hospital
performance rather than individual surgeon performance, and similar
methods of data analysis and determination of outliers. Moreover, SOMIP
allowed individual HA hospitals to benchmark their performance against the
overall HA average.
As all surgical patients have a different health
status, their operation outcomes will likewise differ. Appropriate
adjustment for different patient risks is essential when interpreting
hospital mortality rates. To adjust for different risk factors, over 100
patient risk factors were captured for each enrolled patient. For NSQIP,
one risk-adjustment mortality model was constructed for all operations. In
contrast to NSQIP, separate models were devised for emergency and elective
operations in SOMIP.
Hospital outliers can be identified by O/E ratios
if the confidence interval of the O/E ratio is greater than 1, meaning
that after balancing the different risks of hospital patients, their
clinical outcomes are most likely different from the rest. From the
results of this study, it was encouraging to find a significant trend of
reduction in crude mortality rates and O/E ratios for both elective and
emergency operations at HA hospitals over the past 5 years. According to
the significant reduction in expected odds ratios over the years, this
improvement is genuine and not due to patient selection.
There are several possible reasons behind the
changes: public identification as a poor performer
is a strong incentive for change in HA hospitals;
sharing best practices on perioperative patient
care is an important educational activity that takes
place annually through the SOMIP Forum; the HA
Head Office invests more resources into deficient hospitals; and changing
attitudes towards managing surgical complications
by other colleagues from the intensive care unit are
also helpful. All of these may have contributed to the
change.
Limitations
The SOMIP has a number of limitations. The coverage
of SOMIP is not as complete as that of NSQIP, since many surgical
departments such as orthopaedics and neurosurgery are not included.
Monitoring is done by retrospective annual case collection (from 1 July to
30 June) because it takes 13 months to complete case enrolment and an
additional 3 months for data verification, model building, and statistical
analysis. Because of the small number of events, the current programme is
not able to determine the risk-adjusted outcomes of individual operations
or surgeons. Furthermore, because this programme relies heavily on the HA
central electronic database, it is not easy to extend it to hospitals
without this information infrastructure. Although the trend of reduction
in mortality was statistically significant, we were not able to
demonstrate a causal relationship with SOMIP implementation.
Potential issues with data quality may have
affected the outcomes. In the inter-rater reliability test, the nurse
reviewers were not blinded and this may have caused information bias.
Also, quality of data collection in the initial 2 years may have been
unreliable. As a result, the 5-year cumulative comparison analysis for
emergency and elective operations commenced from 2011/2012, rather than
2008/2009. Furthermore, data definitions are updated regularly in the
operation manual and could have affected the time trend analysis.
Nonetheless, the SOMIP team considered changes in data definitions to be
minor and did not expect a significant impact on the risk models.
Mortality could be influenced by many factors;
ensuring risk adjustments are adequate and appropriate would be a
challenge. Disease factors, stage of disease, and treatment options may
not be fully taken into account by the risk-adjusted models, and data
readiness and availability are further constraints. Surgeon skill and
experience was another aspect that could not be accommodated and was
difficult to adjust for. In the HA, surgical operations are performed by a
team; therefore, it would be difficult to separate individual surgeon
experience and credentials from those of the whole team.
Conclusion
From 2008 to 2016, the HA’s overall crude mortality
rates and risk-adjusted O/E ratios showed a significant trend of reduction
for both emergency and elective operations. The SOMIP enhances
understanding of surgical performance and helps identify areas for
improvement. It allows individual HA hospitals to benchmark their
performance against the overall HA average through risk-adjusted O/E
ratios.
Acknowledgements
We thank the SOMIP Steering Committee of the
Hospital Authority, the Coordinating Committee of Surgery, and the
Biostatistics team of The Chinese University of Hong Kong for their
contributions and helpful comments on this manuscript.
Declaration
The authors have no conflicts of interest to
disclose.
References
1. Lui CW, Boyle FM, Wysocki AP, et al. How
participation in surgical mortality audit impacts surgical practice. BMC
Surg 2017;17:42. Crossref
2. Khuri SF, Daley J, Henderson W, et al.
Risk adjustment of the postoperative mortality rate for the comparative
assessment of the quality of surgical care: results of the National
Veterans Affairs surgical risk study. J Am Coll Surg 1997;185:315-27. Crossref
3. Kiermeier A, Babidge WJ, McCulloch GA,
Maddern GJ, Watters DA, Aitken RJ. National surgical mortality audit may
be associated with reduced mortality after emergency admission. ANZ J Surg
2017;87:830-6. Crossref
4. Hospital Authority Statistical Report
(2015-16). Available from:
http://www.ha.org.hk/haho/ho/stat/HASR15_16.pdf. Accessed 10 Nov 2017.
5. Copeland G, Jones D, Walters M. POSSUM:
A scoring system for surgical audit. Br J Surg 1991;78:355-60. Crossref
6. Copeland G. The POSSUM system of
surgical audit. Arch Surg 2002;137:15-9. Crossref
7. Khuri S, Daley J, Henderson W, et al.
The National VA Surgical Risk Study: risk adjustment for the comparative
assessment of the quality of surgical care. J Am Coll Surg
1995;180:519-31.
8. Daley J, Khuri SF, Henderson W, et al.
Risk adjustment of the postoperative morbidity rate for the comparative
assessment of the quality of surgical care: results of the National
Veterans Affairs surgical risk study. J Am Coll Surg 1997;185:328-40. Crossref
9. Daley J. Validating risk-adjusted
surgical outcomes: site visit assessment of process and structure. J Am
Coll Surg 1997;185:341-51. Crossref
10. Surgical Outcomes Monitoring &
Improvement Program
(SOMIP) report. Volume One: July 2008-June 2009.
Hospital Authority, Hong Kong SAR Government; 2010.
11. Surgical Outcomes Monitoring &
Improvement Program (SOMIP) report. Volume Two: July 2009-June 2010.
Hospital Authority, Hong Kong SAR Government; 2011.
12. Surgical Outcomes Monitoring &
Improvement Program (SOMIP) report. Volume Three: July 2010-June 2011.
Hospital Authority, Hong Kong SAR Government; 2012.
13. Surgical Outcomes Monitoring &
Improvement Program (SOMIP) report. Volume Four: July 2011-June 2012.
Hospital Authority, Hong Kong SAR Government; 2013.
14. Surgical Outcomes Monitoring &
Improvement Program (SOMIP) report. Volume Five: July 2012-June 2013.
Hospital Authority, Hong Kong SAR Government; 2014.
15. Surgical Outcomes Monitoring &
Improvement Program (SOMIP) report. Volume Six: July 2013-June 2014.
Hospital Authority, Hong Kong SAR Government; 2015.
16. Surgical Outcomes Monitoring &
Improvement Program (SOMIP) report. Volume Seven: July 2014-June 2015.
Hospital Authority, Hong Kong SAR Government; 2016.
17. Surgical Outcomes Monitoring &
Improvement Program (SOMIP) report. Volume Eight: July 2015-June 2016.
Hospital Authority, Hong Kong SAR Government; 2017.
18. Ash A, Schwartz M. Evaluating the
performance of risk-adjustment methods: dichotomous variables. In: Iezzoni
L, editor. Risk adjustment for measuring health care outcomes. Ann Arbor,
MI: Health Administration Press; 1994: 313-46.
19. Hall BL, Hamilton BH, Richards K,
Bilimoria KY, Cohen ME, Ko CY. Does surgical quality improve in the
American College of Surgeons National Surgical Quality Improvement
Program: An evaluation of all participating hospitals. Ann Surg
2009;250:363-76. Crossref
20. Cohen ME, Liu Y, Ko CY, Hall BL.
Improved surgical outcomes for ACS NSQIP hospitals over time: evaluation
of hospital cohorts with up to 8 years of participation. Ann Surg
2016;263:267-73. Crossref